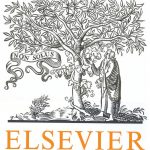
ترجمه مقاله نقش ضروری ارتباطات 6G با چشم انداز صنعت 4.0
- مبلغ: ۸۶,۰۰۰ تومان
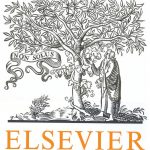
ترجمه مقاله پایداری توسعه شهری، تعدیل ساختار صنعتی و کارایی کاربری زمین
- مبلغ: ۹۱,۰۰۰ تومان
Abstract
Although the conventional Bayesian network classifier (BNC) has been widely reported in the field of fault diagnosis, sometimes, it may not always work well to separate all faults with a single network. Besides, BNC only considers static distribution information but ignores the dynamic information. The dynamic nature in fault data, which is reflected in temporal behaviors of process data, can describe some meaningful underlying fault characteristics. Here, a novel distributed Bayesian network based on slow feature analysis (SFA-DBN) is proposed for fault diagnosis of complex process. The purpose of this work is to improve the performance of BNC for inseparable faults. The first step of this model is to build a global Bayesian network (GBN) to distinguish the inseparable faults from the separable faults. Second, the inseparable faults are divided into several fault subsets on basis of slow feature analysis (SFA) in which, dynamic variations are similar within the same subset while they are significantly different for different subsets. Third, a set of parallel Bayesian networks (PBNs) are designed to construct the distributed BN for fault diagnosis with different BNs for different fault subsets. Two credible criteria are also presented for offline reasonable partition and online correct identification. Finally, Tennessee Eastman (TE) Process verifies the validity of this proposed model.
IV. CONCLUSION
To improve the accuracy of Bayesian network classifier for complex processes, an effective SFA-DBN algorithm is proposed in this paper. By dividing inseparable faults into different subsets and analyzing the dynamic features, the inseparable faults can be well classified by development of PBNs. Furthermore, future work may focus on how to extend the proposed method to trace the fault root variables.