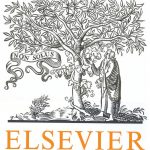
ترجمه مقاله نقش ضروری ارتباطات 6G با چشم انداز صنعت 4.0
- مبلغ: ۸۶,۰۰۰ تومان
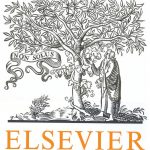
ترجمه مقاله پایداری توسعه شهری، تعدیل ساختار صنعتی و کارایی کاربری زمین
- مبلغ: ۹۱,۰۰۰ تومان
ABSTRACT
This paper presents a new evolutionary cooperative learning scheme, able to solve function approximation and classification problems with improved accuracy and generalization capabilities. The proposed method optimizes the construction of radial basis function (RBF) networks, based on a cooperative particle swarm optimization (CPSO) framework. It allows for using variable-width basis functions, which increase the flexibility of the produced models, while performing full network optimization by concurrently determining the rest of the RBF parameters, namely center locations, synaptic weights and network size. To avoid the excessive number of design variables, which hinders the optimization task, a compact representation scheme is introduced, using two distinct swarms. The first swarm applies the non-symmetric fuzzy means algorithm to calculate the network structure and RBF kernel center coordinates, while the second encodes the basis function widths by introducing a modified neighbor coverage heuristic. The two swarms work together in a cooperative way, by exchanging information towards discovering improved RBF network configurations, whereas a suitably tailored reset operation is incorporated to help avoid stagnation. The superiority of the proposed scheme is illustrated through implementation in a wide range of benchmark problems, and comparison with alternative approaches.
5. Conclusions
This work presents a new method for fully optimizing RBF networks using a cooperative PSO approach. The problem of concurrently optimizing the centers and widths of the basis functions is solved using a specially designed particle encoding, which dismantles the problem in two parts and thus utilizes two distinct swarms. The first swarm is responsible for the selection of the network structure and RBF kernel center coordinates through the NSFM algorithm, whereas the second takes care of width selection by taking into account the nearest neighbor coverage. The two swarms work together towards discovering improved solutions in a cooperative manner, while a specially designed reset operation is introduced to avoid stagnation. The resulting algorithm is evaluated on 9 different benchmarks, covering a range of real world and simulated datasets, which include function approximation and classification problems. For comparison purposes, three additional methodologies are also implemented, namely RBF networks optimized with noncooperative PSO using variable and fixed-width functions, and MFF networks trained using the Levenberg-Marquardt algorithm. Results show that the proposed approach greatly outperforms the MFF networks and the fixed-width method, as far as accuracy is concerned. Moreover, the cooperative algorithm not only provides better and more consistent results in terms of accuracy compared to the non-cooperative variable-width method, but also manages to discover the best solution in less iterations. The superior performance is in most cases combined with smaller network structures.