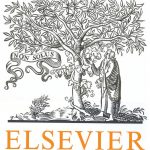
ترجمه مقاله نقش ضروری ارتباطات 6G با چشم انداز صنعت 4.0
- مبلغ: ۸۶,۰۰۰ تومان
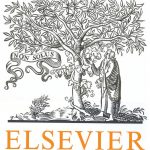
ترجمه مقاله پایداری توسعه شهری، تعدیل ساختار صنعتی و کارایی کاربری زمین
- مبلغ: ۹۱,۰۰۰ تومان
Abstract
Customer engagement is drastically improved through Web 2.0 technologies, especially social media platforms like Twitter. These platforms are often used by organizations for marketing, of which creation of numerous spam profiles for content promotion is common. The present paper proposes a hybrid approach for identifying the spam profiles by combining social media analytics and bio inspired computing. It adopts a modified K-Means integrated Levy flight Firefly Algorithm (LFA) with chaotic maps as an extension to Firefly Algorithm (FA) for spam detection in Twitter marketing. A total of 18,44,701 tweets have been analyzed from 14,235 Twitter profiles on 13 statistically significant factors derived from social media analytics. A Fuzzy C-Means Clustering approach is further used to identify the overlapping users in two clusters of spammers and non-spammers. Six variants of K-Means integrated FA including chaotic maps and levy flights are tested. The findings indicate that FA with chaos for tuning attractiveness coefficient using Gauss Map converges to a working solution the fastest. Further, LFA with chaos for tuning the absorption coefficient using sinusoidal map outperforms the rest of the approaches in terms of accuracy.
Conclusion and Future Research Directions
This study uses a mixed research methodology by combining the insights from social media analytics to model the spammers in Twitter using bio inspired computing. The proposed K-means integrated levy flight algorithm using Sinusoidal map for tuning the absorption coefficient produces the best results in terms of accuracy and a faster convergence rate. The proposed approach gives an accuracy of 97.98% by modeling 13 significant factors after a statistical t-test including emotion diversity, polarity diversity, hashtag frequency, unique words, user @ mentions, lexical diversity, tweet count, follower count, favorite count, friends count, added to lists, following rate and user reputation. In addition to the proposed integrated firefly approach, a Fuzzy C-Means approach is used to identify the overlap among the two spam and authentic fuzzy groups. However, when compared with the proposed approach, the convergence of the Fuzzy C-Means is slower than the proposed approach. The study thus effectively combines relevant factors from user, descriptive and semantic statistics to model the Twitter profiles for detecting social media spam. The proposed approach can prove to be beneficial when organizations seek to gauge the success rate of campaigns, for identifying potential influencers for promotion of content and viral marketing. Our study highlights that analytics driven approach in social media for analyzing spam needs to be developed based on multi-method research methodologies because of the nature of the user generated content as well as the volume of the instances of content creation per content creator