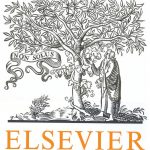
ترجمه مقاله نقش ضروری ارتباطات 6G با چشم انداز صنعت 4.0
- مبلغ: ۸۶,۰۰۰ تومان
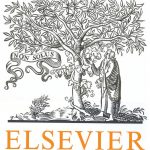
ترجمه مقاله پایداری توسعه شهری، تعدیل ساختار صنعتی و کارایی کاربری زمین
- مبلغ: ۹۱,۰۰۰ تومان
Abstract
Brain-computer interfaces (BCIs) have been investigated in recent years to transfer the brain activities to external devices as rehabilitation tools in clinical trials. Here we present a BCI to detect lower-limb movement intention from electroencephalography (EEG) signals, combining movementrelated cortical potentials (MRCPs) and sensorymotor rhythms (SMRs) with support vector machine (SVM) classification model. We report analysis of the EEG correlates of five healthy subjects while they perform self-paced ankle dorsiflexion. The average detection accuracy was 0.89 ± 0.04, while the latency was - 0.325 ± 0.127 ms with respect to actual movement onset. The combination of these two features has shown significantly better performance (p < 0.01) than the models using either MRCP or SMR. It is also demonstrated that complementary information was employed to boost the detection performance. The proposed paradigm could be further implemented as a brain switch in neurorehabilitation scenarios.
IV. DISCUSSION AND CONCLUSION
In this study, we proposed a BCI framework combining SMR and MRCP for the detection of lower-limb movement intention. Experiment was conducted with healthy subjects demonstrating the applicability of the paradigm with samplebased, BCI command sending, and single-trial performance. In particular, we show the combination of the features extracted from the cortical rhythms and SCP can boost the performance to detect the voluntary movement onset.
Previous studies have described several aspects of the movement onset detection. Upper-limb reaching movement was decoded by Lew et al with an average maximum TPR of 0.76 ± 0.07 and detection latency of 460 ± 85 ms before actual onset [10]. A recent study by Xu et al proposed a detection model with advanced dimensional reduction method and LDA classifier, resulting in a TPR of 0.79 ± 0.12 and latency of 315 ± 165 [16]. A direction comparison of these results could be difficult due to the difference of the protocols and subject variations. Nevertheless, significantly better performances had been achieved with the combined features in all metrics with the same dataset.
In order to build a brain switch for closed-loop BCI, the robust detection is critical. Currently, we obtained promising results with the classification model based on SVM. Further work need to be done with either simplified or more sophisticated signal processing and machine learning techniques. On the other hand, the decoded signal can be used to trigger external devices, e.g., exoskeleton. Further work should be done to provide real-time feedback to the user during the recordings. In this sense, the subjects could learn to modulate their brain modulation for a more effective training.