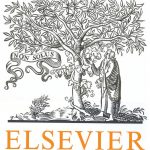
ترجمه مقاله نقش ضروری ارتباطات 6G با چشم انداز صنعت 4.0
- مبلغ: ۸۶,۰۰۰ تومان
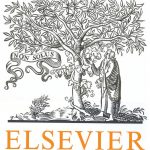
ترجمه مقاله پایداری توسعه شهری، تعدیل ساختار صنعتی و کارایی کاربری زمین
- مبلغ: ۹۱,۰۰۰ تومان
Abstract
Background: Growing concerns about increasing rates of antibiotic resistance call for expanded and comprehensive global monitoring. Advancing methods for monitoring of environmental media (e.g., wastewater, agricultural waste, food, and water) is especially needed for identifying potential resources of novel antibiotic resistance genes (ARGs), hot spots for gene exchange, and as pathways for the spread of ARGs and human exposure. Next-generation sequencing now enables direct access and profiling of the total metagenomic DNA pool, where ARGs are typically identified or predicted based on the “best hits” of sequence searches against existing databases. Unfortunately, this approach produces a high rate of false negatives. To address such limitations, we propose here a deep learning approach, taking into account a dissimilarity matrix created using all known categories of ARGs. Two deep learning models, DeepARG-SS and DeepARGLS, were constructed for short read sequences and full gene length sequences, respectively. Results: Evaluation of the deep learning models over 30 antibiotic resistance categories demonstrates that the DeepARG models can predict ARGs with both high precision (> 0.97) and recall (> 0.90). The models displayed an advantage over the typical best hit approach, yielding consistently lower false negative rates and thus higher overall recall (> 0.9). As more data become available for under-represented ARG categories, the DeepARG models’ performance can be expected to be further enhanced due to the nature of the underlying neural networks. Our newly developed ARG database, DeepARG-DB, encompasses ARGs predicted with a high degree of confidence and extensive manual inspection, greatly expanding current ARG repositories. Conclusions: The deep learning models developed here offer more accurate antimicrobial resistance annotation relative to current bioinformatics practice. DeepARG does not require strict cutoffs, which enables identification of a much broader diversity of ARGs.
Conclusions
Here, a new computational resource for the identification and annotation of ARGs derived from metagenomic data is developed, trained, and evaluated. The deep learning approach proved to be more accurate than the widely used best hit approach and is not restricted to strict cutoffs, thus greatly reducing false negatives and offering a powerful approach for metagenomic profiling of ARGs in environmental compartments. Further, the DeepARG database developed here greatly expands the available ARGs individually available in the currently most widely used CARD, ARDB, and UNIPROT databases, including their existing sequence content and extensive metadata. DeepARG provides a publiclyavailable database structured into a simple category and group hierarchy for each ARG. While DeepARG is not intended to replace CARD or ARDB, in conjunction with deep learning, it aims to improve the ARG annotation by drastically reducing the false negative rate, while maintaining a similarly high true positive rate associated with the traditional best hit approach. The performance of DeepARG highly depends on the quality of the training database. Therefore, the inclusion of new entries based on the alignment’s similarity could integrate genes that have not been validated to produce antibiotic resistance in vivo. However, this in silico gene’s integration is useful to expand the diversity of ARGs, as it is shown by the analysis of novel ARGs where distant genes have been predicted to the correct antibiotic resistance category.