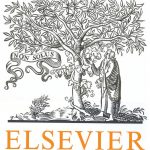
ترجمه مقاله نقش ضروری ارتباطات 6G با چشم انداز صنعت 4.0
- مبلغ: ۸۶,۰۰۰ تومان
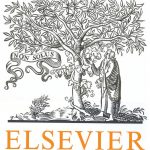
ترجمه مقاله پایداری توسعه شهری، تعدیل ساختار صنعتی و کارایی کاربری زمین
- مبلغ: ۹۱,۰۰۰ تومان
Abstract
Cognitive networks (CNs) are one of the key enablers for the Internet of Things (IoT), where CNs will play an important role in the future internet in several application scenarios, such as healthcare, agriculture, environment monitoring, and smart metering. However, the current low packet transmission efficiency of IoT faces a problem of the crowded spectrum for the rapidly increasing popularities of various wireless applications. Hence, the IoT that uses the advantages of cognitive technology, namely the cognitive radio-based Internet of Things (CIoT), is a promising solution for IoT applications. A major challenge in CIoT is the packet transmission efficiency using CNs. Therefore, a new Q-learning-based transmission scheduling mechanism using deep learning for the CIoT is proposed to solve the problem of how to achieve the appropriate strategy to transmit packets of different buffers through multiple channels to maximize the system throughput. A Markov decision process based model is formulated to describe the state transformation of the system. A relay is used to transmit packets to the sink for the other nodes. To maximize the system utility in different system states, the reinforcement learning method, i.e., the Q learning algorithm, is introduced to help the relay to find the optimal strategy. In addition, the stacked auto-encoders deep learning model is used to establish the mapping between the state and the action to accelerate the solution of the problem. Finally, the experimental results demonstrate that the new action selection method can converge after a certain number of iterations. Compared with other algorithms, the proposed method can better transmit packets with less power consumption and packet loss.
CONCLUSION
In this paper, a relay for transmission packets to the sink of the other nodes is considered in the CIoT. To solve the problem of transmission scheduling in the CIoT, a new Q-learning-based transmission scheduling mechanism using deep learning has been proposed to achieve the appropriate strategy with multiple channels for the cognitive node. In this paper, the reinforcement learning, which is based on the joint action selection criteria of the index value and Q value to balance the action equilibrium problem in exploration and exploitation, is used to solve the MDP problem. Ultimately, it realizes the long-term maximum utility of the system. In addition, the SEA deep learning model is introduced to map between the state and the action. Although the proposed scheme has a lower performance than SI, the algorithm complexity is strongly reduced. The proposed algorithm can work without priori information, which is suitable for practical scenarios. The research in this paper is based on the case of one relay. However, if there are more than one relay scenario, the method to make many relays cooperatively or competitively work can be further studied.