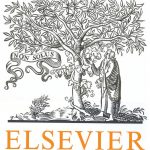
ترجمه مقاله نقش ضروری ارتباطات 6G با چشم انداز صنعت 4.0
- مبلغ: ۸۶,۰۰۰ تومان
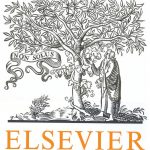
ترجمه مقاله پایداری توسعه شهری، تعدیل ساختار صنعتی و کارایی کاربری زمین
- مبلغ: ۹۱,۰۰۰ تومان
Abstract
Studies that seek to examine the impact of sentiment in financial markets have been affected by inaccurate sentiment measurement and the use of inappropriate data. This study applies state-of-the-art techniques from the domain-general sentiment analysis literature to construct a more accurate decision support system that generates demonstrable improvement in investor sentiment classification performance compared with previous studies. The inclusion of emojis is shown significantly improve sentiment classification in traditional algorithms. Moreover, deep neural networks with domain-specific word embeddings outperform the traditional approaches for the classification of investor sentiment. The approach to sentiment classification outlined in this paper can be applied in future empirical tests that examine the impact of investor sentiment on financial markets.
5. Conclusion, Limitations, and Future Work
The development of an accurate classifier of investor sentiment is required to support decision making in financial markets. Using data from StockTwits, it is shown that MaxEnt and NB outperform SVM despite their simple classification foundation with a strong independence assumption of the features. Moreover, bi-grams and tri-grams robustly boost the classification performance of investor sentiment, capturing long-range dependencies to some extent in the tweets. Although negation is one of the key grammatical rules that inverts the meaning and polarity of a sentence in multiple ways, the implemented negation tagging mechanism does not lead to significant improvement in the performance of classifiers (see Figure 3). Besides, the domain-specific lexicon does not illustrate expected performance in our dataset, confirming its limited ability to capture complex linguistic structures and entities. As discussed in Section 4.1.2, this study reveals that emojis carry very strong discriminative power in the finance context in spite of their domain-specific pattern of usage. Thus, the existence of emojis in the financial texts has contributed substantially to classification performance. In general, deep neural networks significantly outperform traditional methods, depending on the topology and word embeddings (see Figure 7). As we have discussed previously, LSTM and GRU unexpectedly perform better than CNN, although the StockTwits messages are quite short and therefore suitable for CNN to learn local features. LSTM demonstrates robust ability to capture long-term discriminative dependencies without any feature engineering. It is able to focus on the linguistic entities such as emojis, negation, and sarcasm to some degree.