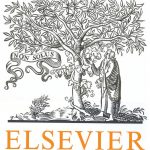
ترجمه مقاله نقش ضروری ارتباطات 6G با چشم انداز صنعت 4.0
- مبلغ: ۸۶,۰۰۰ تومان
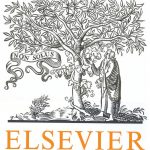
ترجمه مقاله پایداری توسعه شهری، تعدیل ساختار صنعتی و کارایی کاربری زمین
- مبلغ: ۹۱,۰۰۰ تومان
Deep Learning for the IoT
In the era of the IoT, the number of sensing devices that are deployed in every facet of our day-to-day life is enormous. In recent years, many IoT applications have arisen in various domains, such as health, transportation, smart homes, and smart cities [6]. It is predicted by the U.S. National Intelligence Council that, by 2025, Internet nodes will reside in everyday things, such as food packages, furniture, and documents [7]. This expansion of IoT devices, together with cloud computing, has led to creation of an unprecedented amount of data [8], [9]. With this rapid development of the IoT, cloud computing, and the explosion of big data, the most fundamental challenge is to store and explore these volumes of data and extract useful information for future actions [9]. The main element of most IoT applications is an intelligent learning methodology that senses and understands its environment [6]. Traditionally, many machine-learning algorithms were proposed to provide intelligence to IoT devices [10]. However, in recent years, with the popularity of deep neural networks/deep learning, using deep neural networks in the domain of the IoT has received increased attention [6], [11]. Deep learning and the IoT were among the top three technology trends for 2017 announced at Gartner Symposium/ITxpo [12]. This increased interest in deep learning in the IoT domain is because traditional machinelearning algorithms have failed to address the analytic needs of IoT systems [6], which produce data at such a rapid rate and volume that they demand artificial intelligence algorithms with modern data analysis approaches. Depending on the predominant factor, volume or rate, data analytics for IoT applications can be viewed in two main categories: 1) big data analysis and 2) data stream analysis.
Closing Discussion
The ubiquitous deployment of machine learning and artificial intelligence across IoT devices has introduced various intelligence and cognitive capabilities. One may conclude that these capabilities have led to the success of a wide and ever-growing number of applications, such as object/face/speech recognition, wearable devices and biochips, diagnosis software, or intelligent security and preventive maintenance. Developments in other areas, such as humanoid robots, self-driving cars, or smart buildings and cities, will likely revolutionize the way we live in the very near future. This new reality comes with significant advantages but also with many challenges related to the acquisition, processing, storage, exchange, sharing, and interpretation of the continuously growing, overwhelming amount of data generated by the IoT. Up to now, complex applications involving deep neural networks have mainly used the brute force of GPUs for both training and inference. In the last two years, some companies have produced ASICs with better performance and lower power consumption than GPUs. These solutions are suitable for high-performance computing applications, but neither the low flexibility of ASICs nor the high-power consumption of GPUs is suitable for many IoT applications, which demand energy-efficient, flexible embedded systems capable of coping with the increasing diversification of the IoT. In contrast, FPSoC architectures, which include processors and FPGA fabric in the same chip, are a balanced solution to implement machine-learning applications for IoT devices. The latest advancements in FPGA hardware allow a wide range of machine-learning algorithms to be efficiently implemented. FPGAs are very well suited to perform deep neural network inference because of the parallel arrangement of neurons in layers and the type of mathematical functions they have to compute.