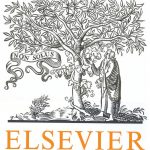
ترجمه مقاله نقش ضروری ارتباطات 6G با چشم انداز صنعت 4.0
- مبلغ: ۸۶,۰۰۰ تومان
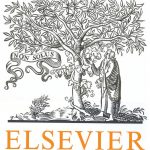
ترجمه مقاله پایداری توسعه شهری، تعدیل ساختار صنعتی و کارایی کاربری زمین
- مبلغ: ۹۱,۰۰۰ تومان
Abstract
In the scope of cognitive radio networks, typical routing protocols avoid areas that are highly congested with primary users, leaving only a small fragment of available links for secondary route construction. In addition, wireless links are prone to channel impairments such as multipath fading which renders the quality of the available links highly fluctuating. In this paper, we propose Undercover : a multi-hop routing protocol for cognitive radio networks in which we integrate the collaborative beamforming technique with layer 3 routing. Specifically, our protocol revisits a fundamental assumption taken by the state of the art routing protocols designed for overlay cognitive radio networks; this assumption is that secondary users cannot use the spectrum when primary users are using it. In Undercover , we allow a group of secondary users, each with a single antenna, to collaborate together and transmit in the regions of primary users activity. This is done through nulling out transmission at primary receivers via beamforming. Moreover, Undercover is designed to enhance the transmission quality at the secondary destinations whenever possible. To account for the excessive levels of interference typically incurred due to cooperative transmissions, we allow our protocol to be interference-aware. Thus, cooperative transmissions are penalized in accordance with the amount of negatively affected secondary flows. We evaluate the performance of our proposed protocol via NS2 simulations which show that our protocol can enhance the network goodput by a ratio reaches up to 250% compared to other state-of-art cognitive routing protocols with minimal added overhead.
7. Conclusion
In this paper, we proposed Undercover , a cross layering routing protocol that integrates physical layer techniques in the routing layer (layer 3). Undercover utilizes cooperative groups and uses beamforming to send data normally even if primary users exist and are active. This property leads to a better packet delivery ratio for Undercover than other protocols. Thus, the ability to send data simultaneously with the primary users opens a new degree of freedom that was not available before. Also, the collaboration between nodes is used to send signals in adhoc networks (the case when no primary users exist) with better qualities. Thus, although our protocol is designed mainly for Cognitive Radio Networks, it proves to be useful also in adhoc networks. Undercover is also designed to be an interference-aware protocol as it takes into consideration the interference that the constructed cooperative groups can inflict on other routes and vice versa. Moreover, search window control heuristic is proposed which aims at shrinking the search space for the potential group members. Evaluating Undercover is done using NS2 where the achieved goodput and the average end-to-end delay are observed. Undercover is compared against CAODV, which is a representative for the geographical protocols, and LAUNCH as an example from the location-aided routing protocols. Undercover achieves a goodput gain that reaches up to 250% compared to other protocols. Also, it shows to have a low overhead and a reasonable end-to-end delay. In addition, the search window control heuristic evaluation shows that it successfully reduces the time of searching for the best group so that the algorithm may be used practically.
Future directions include finding a mathematical model for values in table 2 and a way to improve the group construction time. In this work, we assume that PUs are stationary. One way to extend this work is to assume mobile PUs. One way to accomodate this change is to remember top k groups and choose one of them based on the PUs locations. Moreover, we assume some model of detecting PUs and sensing their activities. Exploring other models of doing this would be a good future direction too.