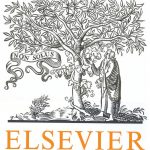
ترجمه مقاله نقش ضروری ارتباطات 6G با چشم انداز صنعت 4.0
- مبلغ: ۸۶,۰۰۰ تومان
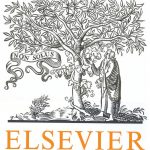
ترجمه مقاله پایداری توسعه شهری، تعدیل ساختار صنعتی و کارایی کاربری زمین
- مبلغ: ۹۱,۰۰۰ تومان
abstract
Markov networks and Bayesian networks are two popular models for classification. Vapnik–Chervonenkis dimension and Euclidean dimension are two measures of complexity of a class of functions, which can be used to measure classification capability of classifiers. One can use Vapnik–Chervonenkis dimension of the class of functions associated with a classifier to construct an estimate of its generalization error. In this paper, we study Vapnik–Chervonenkis dimension and Euclidean dimension of concept classes induced by discrete Markov networks and Bayesian networks. We show that these two dimensional values of the concept class induced by a discrete Markov network are identical, and the value equals dimension of the toric ideal corresponding to this Markov network as long as the toric ideal is nontrivial. Based on this result, one can compute the dimensional value in terms of a computer algebra system directly. Furthermore, for a general Bayesian network, we show that dimension of the corresponding toric ideal offers an upper bound of Euclidean dimension. In addition, we illustrate how to use Vapnik–Chervonenkis dimension to estimate generalization error in binary classification.
6. Discussion
In this paper, we mainly provide three equivalent characterizations of VC dimension of concept classes induced by Markov networks through viewing dimension of a toric ideal as a bridge between VC dimension and Euclidean dimension, and present upper bounds for Euclidean dimension of concept classes induced by Bayesian networks. This concrete connection between algebraic ge ometry and machine learning allows one to compute VC dimension of the concept class induced by a Markov network in terms of a computer algebra system directly. We illustrate the utility of our results in calculating prediction error in binary classification. For Bayesian networks without V-structures, based on levels of each variable, Yang and Wu [37] and Varando et al. [33] offered explicit formula to compute Euclidean dimension and thus VC dimension of induced concept classes. A natural question arises, is there an explicit formula for VC dimension of concept classes induced by Markov networks? For a general Bayesian network ( −→G ,P) (with at least one Vstructure), as far as we know, no other result illustrates the relationship among dimension of the model, VC dimension and Euclidean dimension of concept class induced by ( −→G ,P) except Yang and Wu [38] for the Bayesian networks with less than four variables. As mentioned in Remark 4.1, it seems difficult to tackle the problem given in Yang and Wu [36] using dimension of ideals, that is, whether the VC dimension is equal to the Euclidean dimension remains open.