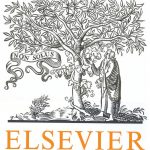
ترجمه مقاله نقش ضروری ارتباطات 6G با چشم انداز صنعت 4.0
- مبلغ: ۸۶,۰۰۰ تومان
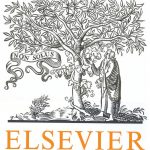
ترجمه مقاله پایداری توسعه شهری، تعدیل ساختار صنعتی و کارایی کاربری زمین
- مبلغ: ۹۱,۰۰۰ تومان
Abstract
We examine the complexity of inference in Bayesian networks specified by logical languages. We consider representations that range from fragments of propositional logic to function-free first-order logic with equality; in doing so we cover a variety of plate models and of probabilistic relational models. We study the complexity of inferences when network, query and domain are the input (the inferential and the combined complexity), when the network is fixed and query and domain are the input (the query/data complexity), and when the network and query are fixed and the domain is the input (the domain complexity). We draw connections with probabilistic databases and liftability results, and obtain complexity classes that range from polynomial to exponential levels; we identify new languages with tractable inference, and we relate our results to languages based on plates and probabilistic relational models.
4. Relational Languages: Inferential, query, and domain complexity
In this section we extend our specification framework so as to deal with relational languages. Such languages have been used in a variety of applications with repetitive entities and relationships [46, 109], as we have alluded to in Section 1. They have roots in general probabilistic logics that mix deterministic knowledge and uncertain reasoning in very flexible, but sometimes too complicated, ways [56]. The more focused interest in extending Bayesian networks with relational constructs has produced an array of practical languages, as we summarize later in Section 7: plates, PRMs, probabilistic logic programs. It is not easy to extract a “common denominator” from these languages. However, with some reflection we see that they all parameterize random variables using relations; they all allow for logical definitions to be mixed with probabilistic assessments; they all resort to the semantics of first-order logic to interpret relations using sets (domains). We capture these common features in Section 4.1; to do so, we use “parvariables” and related techniques introduced by Poole [103], using as much syntax and semantics as possible from first-order logic and in particular from lifted inference techniques [90] (and borrowing some terminology from description logics and logic programming as appropriate). Throughout we resort to the same idea advocated in Section 3: that is, that a model can be specified by a set of probability assessments and a set of logical definitions that belong to a selected logical language. This is exactly the situation in acyclic probabilistic logic programs and many other existing languages, as shown later in Section 7.