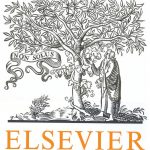
ترجمه مقاله نقش ضروری ارتباطات 6G با چشم انداز صنعت 4.0
- مبلغ: ۸۶,۰۰۰ تومان
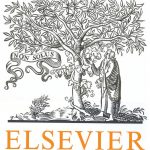
ترجمه مقاله پایداری توسعه شهری، تعدیل ساختار صنعتی و کارایی کاربری زمین
- مبلغ: ۹۱,۰۰۰ تومان
ABSTRACT
Recent years have seen a great deal of work on collection selection. Most collection selection methods use central sample index (CSI) that consists of some documents sampled from each collection as collection description. The limitations of these methods are the usage of ‘flat’ meaning representations that ignore structure and relationships among words in CSI, and the calculation of query-collection similarity metric that ignore semantic distance between query words and indexed words. In this paper, we propose a knowledge based collection selection method (KBCS) to improve collection representation and query-collection similarity metric. KBCS models a collection as a weighted entity set and applies a novel query-collection similarity metric to select highly scored collections. Specifically, in the part of collection representation, contextand structure-based measures are employed to weight the semantic distance between two entities extracted from the sampled documents of a collection. In addition, the novel query-collection similarity metric takes the entity weight, collection size, and other factors into account. To enrich concepts contained in a query, DBpedia based query expansion is integrated. Finally, extensive experiments were conducted on a large webpage dataset, and DBpedia was chosen as the graph knowledge base. Experimental results demonstrate the effectiveness of KBCS.
5. Conclusions and future work
In this paper, we present KBCS that represents collection as a weighted entity set based on context- and structure-based measures, and ranks collections according to a query-collection similarity metric that considers sampling factor, collection entity frequency, collection entity weight, and query entity weight. Context and structure semantic information encoded in DBpedia is exploited, and a DBpedia based query expansion method is also integrated to enrich entities found in query terms. We evaluate KBCS on a large dataset of CW09-CatB that is partitioned into topical collections, and experimental results demonstrate the effectiveness of KBCS.
In the future, we will take other semantic measures (e.g., content-based measure) to weight the entities in CSI accurately, and combine documents score and entities weight to improve the query-collection similarity metric. Furthermore, we will apply contextand structure-based measures to estimate the quality of expanded entities to improve the performance of community search in query expansion, on which the weights assigned to expanded entities can be optimized.