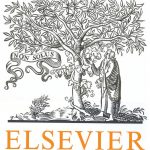
ترجمه مقاله نقش ضروری ارتباطات 6G با چشم انداز صنعت 4.0
- مبلغ: ۸۶,۰۰۰ تومان
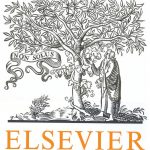
ترجمه مقاله پایداری توسعه شهری، تعدیل ساختار صنعتی و کارایی کاربری زمین
- مبلغ: ۹۱,۰۰۰ تومان
ABSTRACT
Attention Deficit Hyperactive Disorder (ADHD) is one of the most common diseases in school aged children. In this paper, we consider using fMRI data with classification techniques to aid the diagnosis of ADHD and propose a bi-objective ADHD classification scheme based on L1-norm support vector machine (SVM). In our classification model, two objectives, namely, the margin of separation and the empirical error are considered at the same time. Then the normal boundary intersection (NBI) method of Das and Dennis is used to solve the bi-objective optimization problem. A representative nondominated set which reflects the entire trade-off information between the two objectives is obtained. Each representative nondominated point in the set corresponds to an efficient classifier. Finally a decision maker can choose a final efficient classifier from the set according to the performance of each classifier. Our scheme avoids the trial and error process for regularization hyper-parameter selection. Experimental results show that our bi-objective optimization classification scheme for ADHD diagnosis performs considerably better than some traditional classification methods.
Conclusion and future work
In this paper we have addressed the problem of classification of ADHD patients and healthy controls based upon fMRI data. We use the function connectivity information as the feature information for classification. Instead of the traditional weighted sum formulation, we adopt a bi-objective SVM formulation based on L1-norm in which the two objectives are to minimize empirical error and maximize the margin of separation. The normal boundary intersection algorithm is used to generate a representative nondominated set. Each nondominated point in the set corresponds to an efficient classifier for decision makers to choose. Experimental results show that our method can be used for obtaining a good representative set of classifiers in comparison to the traditional SVM formulation with a trade-off parameter C. With bi-objective optimization scheme, an efficient classifier can be selected from the representative set of classifier frontier by decision makers. Comparative analysis shows that our method gives a better performance than L CSVM, L CSV 1 2 M, KNN, AdaBoost, RF, MLP and ELM methods. Further work can be done on the processing of imbalanced data in order to improve the accuracy of classification.