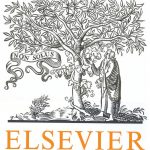
ترجمه مقاله نقش ضروری ارتباطات 6G با چشم انداز صنعت 4.0
- مبلغ: ۸۶,۰۰۰ تومان
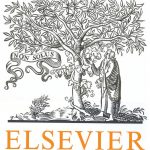
ترجمه مقاله پایداری توسعه شهری، تعدیل ساختار صنعتی و کارایی کاربری زمین
- مبلغ: ۹۱,۰۰۰ تومان
Abstract:
In this paper, a classification method based on deep learning and sparse representation is proposed for gastric slice images. First of all, the convolution features of images are extracted through the convolutional neural network. Then an overcomplete learning dictionary of convolution features can be gained by K-SVD algorithm. Convolution features can obtain their sparse representation through decomposition on the overcomplete dictionary. Finally, a linear SVM classifier is used to classify the sparse representation of convolution features instead of the commonly used nonlinear classifiers, so as to achieve the purpose of classifying gastric slices. Experiments show that using linear SVM reduces the computation cost of classifier and achieves better classification effect than convolutional neural network with sigmoid output layer or SVM with RBF kernel. Therefore, the method proposed in this paper has better classification effect and lower computation cost.
CONCLUSION
In this paper, an image classification method based on deep learning and sparse representation is proposed for the classification of gastric slice images. The convolution features of the images are extracted by CNN. An overcomplete learning dictionary of these features can be then learned through K-SVD algorithm. The convolution features are decomposed on overcomplete dictionary to get their sparse representation. Finally, the best classification result can be obtained by only using linear SVM, not nonlinear. This method reduces the computational complexity of the classifier while obtaining the best classification effect, which may make the classification method work in real time.