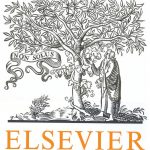
ترجمه مقاله نقش ضروری ارتباطات 6G با چشم انداز صنعت 4.0
- مبلغ: ۸۶,۰۰۰ تومان
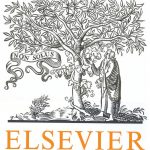
ترجمه مقاله پایداری توسعه شهری، تعدیل ساختار صنعتی و کارایی کاربری زمین
- مبلغ: ۹۱,۰۰۰ تومان
abstract
Understanding the functions of the visual system has been one of the major targets in neuroscience for many years. However, the relation between spontaneous brain activities and visual saliency in natural stimuli has yet to be elucidated. In this study, we developed an optimized machine learning-based decoding model to explore the possible relationships between the electroencephalography (EEG) characteristics and visual saliency. The optimal features were extracted from the EEG signals and saliency map which was computed according to an unsupervised saliency model (Tavakoli and Laaksonen, 2017). Subsequently, various unsupervised feature selection/extraction techniques were examined using different supervised regression models. The robustness of the presented model was fully verified by means of ten-fold or nested cross validation procedure, and promising results were achieved in the reconstruction of saliency features based on the selected EEG characteristics. Through the successful demonstration of using EEG characteristics to predict the real-time saliency distribution in natural videos, we suggest the feasibility of quantifying visual content through measuring brain activities (EEG signals) in real environments, which would facilitate the understanding of cortical involvement in the processing of natural visual stimuli and application developments motivated by human visual processing.
5. Discussions and conclusion
In the present study, we explored the possible relationship between the EEG activities and saliency embedded in visual stimuli, and developed an optimized decoding model that well predicted the visual saliency distribution based on the EEG characteristics. In accordance with the results obtained in the Steady State Visual Evoked Potentials (SSVEP) study (Uribe et al., 2014), placements of a total of 19 EEG electrodes were first chosen and the corresponding frequency domain features were extracted. Subsequently, various machine learning models together with different feature selection/extraction methods were implemented to find the key EEG characteristics that are evoked, which thus would be involved in the visual attention and have a strong relationship with the saliency distribution. Finally, KPCA+KRR, an optimal decoding model under a combination of unsupervised feature extraction and supervised regression, was presented to estimate the changes of visual saliency based on the extracted EEG characteristics. Very promising prediction/reconstruction performance was achieved in the CV procedure and the generality and adaptivity of the present pipeline were also demonstrated. The best prediction performance was: PCC = 0.94 and NMSE = 0.85. We confirmed through a nested CV that this promising performance was minimally overestimated.
Considering to capture the rich frequency features and to maintain more details in EEG dynamics, we set the moving window size at 2s to perform continuous mapping between EEG signals and visual saliency. We believe the visual latency between the appearance of a video frame and the evoked response in the EEG signals could be neglected. One of the reasons is that the most responsible regions for visual saliency, occipital regions like V1 and V2/3, showed good agreement with existing neuroscience studies (de Graaf, Koivisto, Jacobs, & Sack, 2014; Emmanouil, Avigan, Persuh, & Ro, 2013; Koivisto, Mäntylä, & Silvanto, 2010). The latency from visual stimuli to be processed in such early/early-middle visual areas is known as 100∼200 ms, which is much shorter than the moving window size (2s) used in our decoding model. Also, if considering the most famous evoked EEG, P300, it still has 300 ms latency from the onset of visual stimuli. We actually slightly shifted the EEG signals with 200 ms and did some regression experiments, but the results were almost the same. So, the visual latency could be negligible in this study.