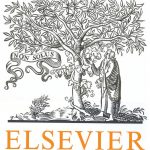
ترجمه مقاله نقش ضروری ارتباطات 6G با چشم انداز صنعت 4.0
- مبلغ: ۸۶,۰۰۰ تومان
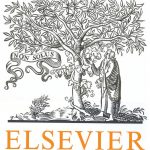
ترجمه مقاله پایداری توسعه شهری، تعدیل ساختار صنعتی و کارایی کاربری زمین
- مبلغ: ۹۱,۰۰۰ تومان
Abstract
Optimization problem often require the use of optimization methods that permit the minimization o maximization of certain objective functions. ccasionally, the problems that must be optimize are not linear or polynomial they cannot be precisely resolved and they must be approximated n these cases, it is necessary to apply heuristics which are able to resolv these kind of problems. Some algorithms linearize the restrictions and objective functions at a specific point of the space by applying derivatives and partial derivatives for some cases, while in other cases evolutionary algorithms are used to approximate the solution. his work proposes the use of artificial neural networks to approximate the objective function in optimization problems to make it possible to apply other techniques to resolve the problem. The objective function is approximate by a non linear regression that can be used to resolve a optimization problem. The derivate of the new objective function should be polynomial so that the solution of the optimization problem can be calculated.
5. Results and conclusions
In order to analyze the performance of the proposal, we analyzed different optimization problems and compared the predicted and optimal values in the system. The tests were made with a neural networks tool developed by our research group and the Mathematica program. Mathematica was used to solve the equations after defining the approximation with a multilayer perceptron. The dataset used to train the neural network is generated according to the domain of the variables. It contains the input variables in the objective function and the output in the objective function obtained for these values. The domain of the variables is defined in the constraints of the optimization problem.
The first test was to analyze the performance of the system with a simple optimization problem. It was a linear function that was approximated with a multilayer perceptron, which activates functions in the hidden and output linear layers.