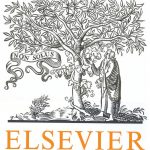
ترجمه مقاله نقش ضروری ارتباطات 6G با چشم انداز صنعت 4.0
- مبلغ: ۸۶,۰۰۰ تومان
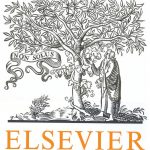
ترجمه مقاله پایداری توسعه شهری، تعدیل ساختار صنعتی و کارایی کاربری زمین
- مبلغ: ۹۱,۰۰۰ تومان
Abstract
With the increasing number of satellite, the satellite control resource scheduling problem (SCRSP) has been main challenge for satellite networks. SCRSP is a constrained and large scale combinatorial problem. More and more researches focus on how to allocate various measurement and control resources effectively to ensure the normal running of the satellites. However, the sparse solution space of SCRSP leads its complexity especially for traditional optimization algorithms. As the validity of ant colony optimization (ACO) has been shown in many combinatorial optimization problems, a simple ant colony optimization algorithm (SACO) to solve SCRSP is presented in this paper. Firstly, we give a general mathematical model of SCRSP. Then, a optimization model, called conflict construction graph, based on visible arc and working period is introduced to reduce workload of dispatchers. To meet the requirements of TT & C network and make the algorithm more practical, we make the parameters of SACO as constant, which include the bounds, update and initialization of pheromone. The effect of parameters on the algorithm performance is studied by experimental method based on SCRSP. Finally, the performance of SACO is compared with other novel ACO algorithms to show the feasibility and effectiveness of improvements.
5 Conclusion
How to effectively schedule the resource to ensure the normal operation of orbiting satellites is one of the main task for satellite networks. The model will be different due to the different constraints and different optimization objectives. It is one of the complexities of the SCRSP. We discuss the general constraints of SCRSP and get the optimization model based on visible arc to reduce the workload of dispatchers. Then we get a novel ant colony optimization based on MMAS to get the optimal solution. Two improvements have done in SACO. First, update amount of pheromone is changed to a constant related to ρ and τmax. Second, the pheromone is initialized to a constant. Then a new pheromone model is generated by combining the two improvements and the constant pheromone bounds. There are two benefits to use this model. On one hand, the degree of parameters coupling is reduced. On the other hand, SACO can be easily masted by the crew of station network. To present the feasibility and effectiveness of the novel work, some simulation experiments on practical instances. Experimental results showed that the performance of SACO is better than that of MMAS and MSCRSP-ACO.
Compared with the evolution process of SACO and MSCRSP-ACO we can find that sustainability of searchability is also important to algorithm. It relates to another important content for an optimization algorithm, that is, keeping the balance between exploration and exploitation. we find that using the pheromone model in SACO is helpful to keep this balance in some extent. But we cannot clearly explain the reason. Furthermore, how to decide the key factor and minor factor for the performance of SACO is also a question. They may be one of future work for us. In addition, how to use the problem characteristics to design more effective algorithm including the algorithm selecting and parameter setting is another future work. Last, how to build a united platform to compare the performance of different metaheuristic algorithms is also important work in the future.