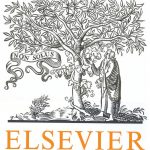
ترجمه مقاله نقش ضروری ارتباطات 6G با چشم انداز صنعت 4.0
- مبلغ: ۸۶,۰۰۰ تومان
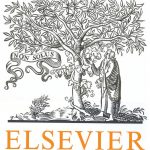
ترجمه مقاله پایداری توسعه شهری، تعدیل ساختار صنعتی و کارایی کاربری زمین
- مبلغ: ۹۱,۰۰۰ تومان
Abstract:
In this work, an innovative approach to exploit the true potentials of machine learning (ML) by the financial industry is presented; using ML technology not as a source of investment ideas but as a consultant for trading decisions. In particular, the artificial intelligent risk management system (AIRMS) is presented that introduces one of the first efforts in the literature to utilize supervised ML as a risk management tool. Two AIRMSs systems are developed based on two well-known ML algorithms, i.e. artificial neural networks and decision trees. These two systems are applied into the five major currency pairs (FOREX) using signals obtained from an existing technical breakout trading strategy introduced in previous study by the authors, covering a seven-year period (2010-2016). Technical indicators and times series of the past entry points feed AIRMS in order to classify produced signals from the trading strategy into two classes: profitable and not. Constructing new portfolios using signals classified only as profitable resulted in an increased profit of more than 50% compared to the original ones. In this work, technical improvements are also proposed on the application of ML algorithms to financial data related to evaluation metrics and smoothing inputs. The obtained results revealed that the two AIRMSs can achieve impressive improvements to the performance of already profitable portfolios and proved that using ML to build risk management tools is very promising.
5. CONCLUSION
The major achievement of this study was the successful application of two machine learning models for risk management purposes; resulting in the development of an artificial intelligent risk management system (AIRMS). Decision trees (DT) and artificial neural networks (ANN) are the two models that were used in this work. In previous work (Chandrinos and Lagaros, 2018), the authors proposed two profitable currency portfolios (labeled as EWP2 and KCP2) that were based on the optimized channel MRB strategy. These two portfolios are constituted by five currencies exchange rates (GBP/USD, USD/JPY, EUR/USD, GBP/JPY and EUR/JPY) and their returns refer to a seven-year period, from the year 2010 to 2016.
The main target of the developed AIRMS was to improve the performance of the two portfolios not by generating new profits but preventing them from losses. Therefore, AIRMS was applied to the optimized trading strategy in order to recognize which signals will be profitable and which ones will not, transforming the profitable ones into trades. For both risk management systems developed in the current study (i.e. DT and ANN based ones, labeled as AIRMS-DT and AIRMS-ANN, respectively) the dynamic moving window was used in order to train, test and finally predict the quality of the produced signals of the next year. Worth mentioning that the way that the two machine learning models were calibrated is crucial for the results produced and that the two systems succeeded to provide significant amelioration on the performance of both EWP2 and KCP2 currency portfolios, resulting into improved portfolios that can be even more attractive to potential investors. The two systems not only increased the profitability of both portfolios but also mainly managed to further strengthen their sharpe ratio by reducing the standard deviation.