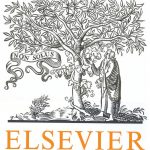
ترجمه مقاله نقش ضروری ارتباطات 6G با چشم انداز صنعت 4.0
- مبلغ: ۸۶,۰۰۰ تومان
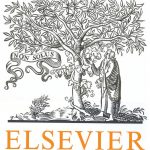
ترجمه مقاله پایداری توسعه شهری، تعدیل ساختار صنعتی و کارایی کاربری زمین
- مبلغ: ۹۱,۰۰۰ تومان
Anomaly intrusion detection in big data environments calls for lightweight models that are able to achieve real-time performance during detection. Abstracting audit data provides a solution to improve the efficiency of data processing in intrusion detection. Data abstraction refers to abstract or extract the most relevant information from the massive dataset. In this work, we propose three strategies of data abstraction, namely, exemplar extraction, attribute selection and attribute abstraction. We first propose an effective method called exemplar extraction to extract representative subsets from the original massive data prior to building the detection models. Two clustering algorithms, Affinity Propagation (AP) and traditional k-means, are employed to find the exemplars from the audit data. k-Nearest Neighbor (k-NN), Principal Component Analysis (PCA) and one-class Support Vector Machine (SVM) are used for the detection. We then employ another two strategies, attribute selection and attribute extraction, to abstract audit data for anomaly intrusion detection. Two http streams collected from a real computing environment as well as the KDD’99 benchmark data set are used to validate these three strategies of data abstraction. The comprehensive experimental results show that while all the three strategies improve the detection efficiency, the AP-based exemplar extraction achieves the best performance of data abstraction.
1 Introduction
The importance of computer network security is growing with the pervasive involvement of computers in people’s daily lives and in business processes within most organizations. As an important technique in the defense-indepth network security framework, intrusion detection has become a widely studied topic in computer networks in recent years.
In general, the techniques for intrusion detection can be categorized as signature-based detection and anomaly detection. Signature-based detection (e.g., Snort [31]) relies on a database of signatures from known malicious threats. Anomaly detection, on the other hand, defines a profile of a subject’s normal activities and attempts to identify any unacceptable deviation as a potential attack. Typically, machine learning techniques are used to build normal profiles of a subject. Any observable behavior of a system, such as a network’s traffic [13,19], a computer host’s operating system [11,36] or a mobile application [2,39], can be used as the subject information.
6 Concluding remarks
The amount of data in anomaly intrusion detection is becoming increasingly massive in current computing environments. Building a lightweight model for anomaly intrusion detection to achieve real-time detection therefore becomes an important challenge. In this paper, we abstract big audit data by finding a small set of exemplars from a large set of original data. An exemplar is nicely representative of other data items. Exemplars are identified among data items and clusters of data items are formed around these exemplars. The exemplars are then fed as data input for training the detection models. This method improves detection efficiency for two reasons: first, only a smaller set of data needs to be processed for the training, and second, the detection process only needs to be based on a compressed model. For a comparative view of different strategies of data abstraction in intrusion detection, in this paper we also introduced Information Gain based attribute selection and PCA based attribute abstraction for anomaly detection.
6. نتیجه گیری ها
مقدار داده ها در تشخیص نفوذ ناهنجاری در محیط های رایانشی فعلی به سرعت در حال گسترش است. بنابراین ایجاد یک مدل سبک وزن برای تشخیص نفوذ ناهنجاری به منظور دستیابی به شناسایی لحظه ای به چالش مهمی تبدیل شده است. ما در این مقاله داده های بزرگ را با یافتن مجموعه کوچکی از نمونه ها از مجموعه بزرگی از داده های اصلی خلاصه می کنیم. یک نمونه به خوبی نشان دهنده آیتم های دیگر داده ها است. نمونه ها در بین آیتم های داده شناسایی می شوند و خوشه های مربوط به آیتم های داده در اطراف این نمونه ها شکل می گیرند. سپس این نمونه ها به عنوان ورودی داده برای آموزش مدل های شناسایی مورد استفاده قرار می گیرند. این روش سبب بهبود کارآیی به دو دلیل می شود: اول، تنها یک مجموعه کوچکتر از داده ها برای پردازش مورد نیاز است و دوم، فرآیند تشخیص تنها می بایست براساس یک مدل فشرده انجام شود. در این مقاله برای نمایش قیاسی از استراتژی های مختلف خلاصه سازی داده ها در تشخیص نفوذ، انتخاب ویژگی مبتنی بر بهره اطلاعات و خلاصه سازی ویژگی مبتنی بر PCA برای تشخیص ناهنجاری معرفی شده است.