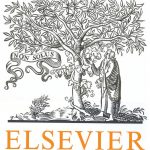
ترجمه مقاله نقش ضروری ارتباطات 6G با چشم انداز صنعت 4.0
- مبلغ: ۸۶,۰۰۰ تومان
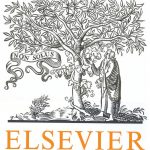
ترجمه مقاله پایداری توسعه شهری، تعدیل ساختار صنعتی و کارایی کاربری زمین
- مبلغ: ۹۱,۰۰۰ تومان
۱ مقدمه
۲ علامتگذاریها و کارهای وابسته
۱ ۲ فهرست علامات
۲ ۲ ۲DLDA
۳ ۲ SVM خطی
۴ ۲ STM
۳ SVM چند خطی چند مرتبه ای
۱ ۳ رابطه بین SVM و STM
۲ ۳ محدودیت های چند خطی چند مرتبه ای
۳ ۳ فرمول بندی (فرمولاسیون)
۴ ۳ راه حل
۴ تحلیل عملکرد
۱ ۴ تحلیل همگرایی
۲ ۴ مقدار دهی اولیه
۳ ۴ پیچیدگی محاسباتی
۴ ۴ تعیین پارامتر
۵ آزمایشات
۱ ۵ شرح داده ها
۲ ۵ رفتار همگرایی
۳ ۵ نتایج دسته بندی
۴ ۵ مقدار دهی های اولیه مختلف
۵ ۵ پیچیدگی محاسباتی
۶ نتیجه گیری
Matrices, or more generally, multi-way arrays (tensors) are common forms of data that are encountered in a wide range of real applications. How to classify this kind of data is an important research topic for both pattern recognition and machine learning. In this paper, by analyzing the relationship between two famous traditional classification approaches, i.e., SVM and STM, a novel tensor-based method, i.e., multiple rank multi-linear SVM (MRMLSVM), is proposed. Different from traditional vector-based and tensor based methods, multiple-rank left and right projecting vectors are employed to construct decision boundary and establish margin function. We reveal that the rank of transformation can be regarded as a tradeoff parameter to balance the capacity of learning and generalization in essence. We also proposed an effective approach to solve the proposed non-convex optimization problem. The convergence behavior, initialization, computational complexity and parameter determination problems are analyzed. Compared with vector-based classification methods, MRMLSVM achieves higher accuracy and has lower computational complexity. Compared with traditional supervised tensor-based methods, MRMLSVM performs better for matrix data classification. Promising experimental results on various kinds of data sets are provided to show the effectiveness of our method.