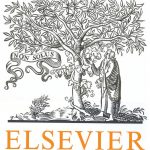
ترجمه مقاله نقش ضروری ارتباطات 6G با چشم انداز صنعت 4.0
- مبلغ: ۸۶,۰۰۰ تومان
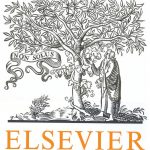
ترجمه مقاله پایداری توسعه شهری، تعدیل ساختار صنعتی و کارایی کاربری زمین
- مبلغ: ۹۱,۰۰۰ تومان
Many researchers have argued that data mining can improve the performance of intrusion detection system. So as one of important techniques of data mining, clustering is an important means for intrusion detection. Due to the disadvantages of traditional clustering methods for intrusion detection, this paper presents a graph-based intrusion detection algorithm by using outlier detection method that based on local deviation coefficient (LDCGB). Compared to other intrusion detection algorithm of clustering, this algorithm is unnecessary to initial cluster number. Meanwhile, it is robust in the outlier’s affection and able to detect any shape of cluster rather that the circle one only. Moreover, it still has stable rate of detection on unknown or muted attacks. LDCGB uses graph-based cluster algorithm (GB) to get an initial partition of data set which is depended on parameter of cluster precision rather than initial cluster number. On the other hand, because of this intrusion detection model is based on mixed training dataset, so it must have high label accuracy to guarantee its performance. Therefore, in labeling phrase, the algorithm imposes outlier detection algorithm of local deviation coefficient to label the result of GB algorithm again. This measure is able to improve the labeling accuracy. The detection rate and false positive rate are obtained after the algorithm is tested by the KDDCup99 data set. The experimental result shows that the proposed algorithm could get a satisfactory performance.
بسیاری از محققین این گونه استدلال کرده اند که داده کاوی می تواند عملکرد سیستم تشخیص نفوذ را بهبود بخشد. بنابراین به عنوان یکی از تکنیک های مهم داده کاوی، خوشه بندی یک وسیله مهم برای تشخیص نفوذ محسوب می شود.به خاطر معایب روشهای خوشه بندی سنتی تشخیص نفوذ، مقاله حاضر الگوریتم تشخیص نفوذ مبتنی بر گراف با استفاده از روش تشخیص نمونه دورافتاده مطرح می کند که براساس ضریب انحراف محلی عمل می کند. در مقایسه با سایر الگوریتم های تشخیص نفوذ خوشه بندی، این الگوریتم برای تعداد خوشه های اولیه ضروری نیست. ضمناً، در تاثیرگذاری بر نمونه دورافتاده پایدار عمل کرده و توانایی تشخیص هر شکل خوشه به جای تنها دایره را دارد. به علاوه،دارای نرخ تشخیص ثابت حملات نامعلوم یا خاموش و آرام می باشد. LDCGB از الگوریتم خوشه بندی مبتنی برگراف (GB) برای دستیابی به یک پارتیشن اولیه از مجموعه داده ها استفاده می کند که وابسته به پارامتر دقت خوشه می باشد نه تعداد خوشه. از طرف دیگر، به این دلیل که این مدل تشخیص نفوذ براساس مجموعه داده آموزشی مختلط عمل می کند، در نتیجه برای تضمین عملکردش، بایستی دارای دارای صحت بالای برچسب باشد. بنابراین، در عبارت برچسب گذاری، الگوریتم، الگوریتم تشخیص نمونه دور افتاده ضریب انحراف محلی برای برچسب گذاری نتیجه الگوریتم GB را تحمیل می کند. این معیار توانایی بهبود صحت برچسب گذاری را دارد. نرخ تشخیص و نرخ مثبت کاذب بعد از تست الگوریتم توسط مجموعه داده بدست می آیند. نتیجه آزمایش نشان می دهد الگوریتم پیشنهادی قادراست به عملکرد رضایت بخشی دست یابد.