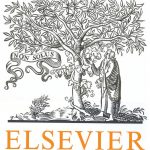
ترجمه مقاله نقش ضروری ارتباطات 6G با چشم انداز صنعت 4.0
- مبلغ: ۸۶,۰۰۰ تومان
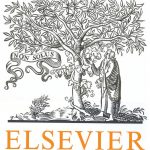
ترجمه مقاله پایداری توسعه شهری، تعدیل ساختار صنعتی و کارایی کاربری زمین
- مبلغ: ۹۱,۰۰۰ تومان
The standard particle filter (PF) algorithm has the issue of particle diversity loss caused by particle degradation and resampling, which makes it impossible for particle samples to accurately represent the true distribution of state probability density function. Particle swarm optimization (PSO) algorithm can effectively improve the particle degradation problem of particle filter namely, PSO-PF, but its fitness function is greatly affected by the variance of measurement noise, and is easy to fall into local optimal, which greatly limits the filtering accuracy. Therefore, this paper proposes an algorithm that combines genetic algorithm (GA) and PSO algorithm to improve particle filtering, namely, GA-PSO-PF. This algorithm combines the fast convergence speed of particle swarm optimization with the strong global searching ability of genetic algorithm to increase the diversity of particles while ensuring the effectiveness of superior particles, and improve the speed and accuracy of finding the optimal solution. Experimental results show that the filtering performance of the proposed algorithm is better than PF and PSO-PF, and the positioning and tracking accuracy is improved by 54.44% compared with PF and 27.20% compared with PSO-PF.
1. Introduction
Particle filtering (PF), also known as the Sequential Monte Carlo method, the core idea of which is to approximate the probability density function of the system with some discrete random sampling points to obtain the minimum variance estimation of the state[1]. Not limited by the linear system model and Gaussian distribution hypothesis, it has been widely used in computer vision [2], image processing [3] and radar target tracking [4] fields. The schematic diagram of the algorithm is shown in Figure 1. However, the standard particle filter algorithm has the problem of particle degradation [5], some researchers have proposed a series of improvement methods. Rudolph et al. [6] introduced the Unscented Kalman Filter (UKF) method into Particle filtering and proposed the Unscented Particle Filter (UPF). Many researchers have also used some intelligent algorithms to optimize the particle filter algorithm[7][8][9].
الگوریتم فیلتر ذرات (PF) استاندارد مسئله فقدان تنوع ذرات ناشی از تخریب و نمونه برداری ذرات است که این امر برای نمونه های ذرات به طور دقیق توزیع صحیح و تابع چگالی احتمال حالت را غیرممکن می سازد. الگوریتم بهینه سازی ازدحام ذرات (PSO) می تواند به طور مؤثر مشکل تخریب ذرات از فیلتر ذرات یعنی PSO-PF را بهبود بخشد، اما تابع سازگاری آن تا حد زیادی تحت تأثیر واریانس نویز اندازه گیری است و به آسانی به بهینه محلی تنزل می یابد که تا حد زیادی دقت فیلتر کردن را محدود می سازد. بنابراین، این مقاله الگوریتمی را پیشنهاد می دهد که الگوریتم ژنتیک (GA) و الگوریتم PSO را ترکیب می کند تا فیلتر کردن ذرات یعنی GA-PSO-PF را بهبود بخشد. این الگوریتم سرعت همگرایی سریع بهینه سازی ازدحام ذرات را با قابلیت جستجوی قوی سراسری الگوریتم ژنتیک ترکیب می کند تا تنوع ذرات را ضمن تضمین اثربخشی ذرات برتر افزایش دهد و سرعت و دقت یافتن جواب بهینه را بهبود بخشد. نتایج تجربی نشان می دهد که عملکرد فیلتر کردن الگوریتم پیشنهادی بهتر از PF و PSO-PF است، و دقت موقعیت یابی و ردیابی در مقایسه با PF 54.44% و درمقایسه با PSO-PF 27.20% بهبود یافته است.
1. مقدمه
ایده اصلی فیلتر کردن ذرات (PF) که به عنوان روش ترتیبی مونت کارلو نیز شناخته شده است، تقریب تابع چگالی احتمال سیستم با برخی از نقاط نمونه برداری تصادفی گسسته جهت کسب مینیمم برآورد واریانس حالت است ]1[. به مدل خطی سیستم و فرضیه توزیع گاوسی محدود نمی شود، و به طور گسترده در زمینه های بینایی کامپیوتر [2]، پردازش تصویر [3] و ردیابی رادار هدف [4] به کار رفته است. نمودار شماتیک الگوریتم در شکل 1 نشان داده شده است. با اینحال، الگوریتم فیلتر ذرات استاندارد دارای مشکل تخریب ذرات است [5]، برخی از پژوهشگران مجموعه ای از روش های بهبود را پیشنهاد داده اند. رودولف و همکاران ]6[ روش فیلتر کالمن بی اثر (UKF) را برای فیلتر کردن ذرات معرفی کردند و فیلتر ذرات بی اثر (UPF) را پیشنهاد دادند. بسیاری از پژوهشگران نیز برخی الگوریتم های هوشمند را جهت بهینه سازی الگوریتم فیلتر ذرات به کار برده اند [7] [8] [9].