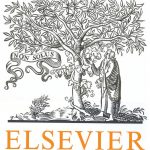
ترجمه مقاله نقش ضروری ارتباطات 6G با چشم انداز صنعت 4.0
- مبلغ: ۸۶,۰۰۰ تومان
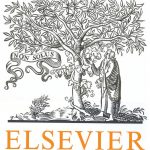
ترجمه مقاله پایداری توسعه شهری، تعدیل ساختار صنعتی و کارایی کاربری زمین
- مبلغ: ۹۱,۰۰۰ تومان
Abstract
Environmental science has developed a diverse set of theories, analytical tools and models to understand and predict ecological responses to human impacts. We review recent innovations in the family of methods used to forecast global environmental change, and offer constructive critiques of five common approaches: phenomenological projections, storyline scenarios, integrated assessment models, decomposition-identity approaches, and global climate simulations. Overall, there is a lack of coherent, empirically based validation for many methods and their assumptions, and only partial incorporation of underlying uncertainties in both parameter estimates and interrelationships of model components. The greatest improvements in global environmental forecasting will likely come from a more systemic approach to quantifying the aggregate socio-economic drivers of the agents of change, along with better integration of multi-disciplinary approaches.
Conclusions
A range of useful methods has been developed to project global change. Yet, as reviewed above, there are clearly limitations with all of these lines of attack. Perhaps most pressingly, global-change science still lacks a coherent, empirically based, statistically robust, and transparent methodology to understand and forecast human drivers of land-use change (and associated impacts) and in turn connect thisto biodiversity responses atregional to globalscales. This constrains our understanding of both the long-term prospects of biodiversity change and of what interventions might be most effective. At higher levels of aggregation, patterns in consumption and use of technology over time and between countries and regions constitute perhaps the most readily identifiable and consistent bases for projecting change. To increase confidence in our representations of the future, we must seek broad expert elicitation (for proper representation of different disciplinary perspectives) and ensure that models (and assumptions) are validated against robust historical data on key uncertainties, such as rates of technology uptake and barriers to deployment. Confidence in the likelihood of scenarios can be enhanced by analysis of the short-term impact of already announced government policy targets (assuming they are implemented in full, e.g., IEA, 2010) or by reference to the envisaged goals from organizations or businesses with a strong track record at delivery (Chang & Baek, 2010; Smil, 2010; Nicholson, Biegler, & Brook, 2011). Quantitative tools like multi-criteria decisionmaking analysis, decomposition and input-output models (Rose & Casler, 1996; Hong, Bradshaw, & Brook, 2013) offer a particularly useful pathway for ensuring high levels of robustness and openness in such validation. Models should also be tested repeatedly against real-world data on patterns and trends – just like hypotheses – to learn from their failures as much as their successes (Brook, Burgman, Akcakaya, O’grady, & Frankham, 2002; Grimm et al., 2005).