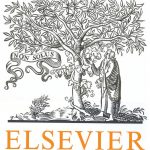
ترجمه مقاله نقش ضروری ارتباطات 6G با چشم انداز صنعت 4.0
- مبلغ: ۸۶,۰۰۰ تومان
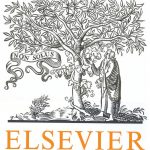
ترجمه مقاله پایداری توسعه شهری، تعدیل ساختار صنعتی و کارایی کاربری زمین
- مبلغ: ۹۱,۰۰۰ تومان
Introduction
Different cultures
The culture and ways in which the statistical community thinks of analyzing and interpreting data have been rapidly evolving in recent years, with the machine learning and signal processing communities having a fundamental impact on the rate and direction of this evolution. To set the stage for this discussion article, it is helpful to first comment on the culture and background of the machine learning and statistical communities. These comments are meant to give a “cartoon” of a complex reality, with this cartoon helpful as a starting point for discussion. Machine learning (ML) community: tends to have its roots in engineering, computer science, and to a certain extent neuroscience – growing out of artificial intelligence (AI). The main publication outlets tend to be peer-reviewed conference proceedings, such as Neural Information Processing Systems (NIPS), and the style of research is very fast paced, trendy, and driven by performance metrics in prediction and related tasks. One measure of “trendiness” is the fact that there is a strong auto-correlation in the main focus areas that are represented in the papers accepted to NIPS and other top conferences. For example, in the past several years much of the focus has been on deep neural network methods. The ML community also has a tendency towards marketing and salesmanship, posting talks and papers on social media and attempting to sell their ideas to the broader public. This feature of the research seems to reflect a desire or tendency to want to monetize the algorithms in the near term, perhaps leading to a focus on industry problems over scientific problems, where the road to monetization is often much longer and less assured. ML marketing has been quite successful in recent years, and there is abundant interest and discussion in the general public about ML/AI, along with increasing success in start-ups and industrial sector high paying jobs partly fueled by the hype.
Discussion
In this short discussion article, I have attempted to provide a brief overview of what I see as the role of statistics in the era of big data – the theme of this special journal issue. I view myself as a statistician with an active interest and research agenda focused on developing and applying machine learning methods. My own research tends to be fundamentally application-driven, and I want to develop practically useful methods that can lead to new scientific insights and that can ideally inform policy. I work closely with scientists in a wide variety of research areas ranging from neuroscience to genomics to epidemiology to ecology. In scientific applications collecting high-dimensional and complex data, there is a fundamental danger to applying current ML-style statistical methods. These include the lack of uncertainty quantification, the inability to provide a warning that we are being too ambitious and should attempt “coarser scale” inferences, and the lack of accounting for selection bias and the sampling frame under which the data were developed. “Modern” statistical theory and methods essentially take a ML mindset to attacking high-dimensional data problems, and hence also do not currently provide much in the way of useful solutions to these pressing problems. I am hoping that this article and the corresponding discussions in this special issue stimulate much more of a focus on developing statistically well grounded methodology for reliably and reproducibly conducting scientific inferences and making policies on the basis of “big data.” Such developments will likely require a close collaboration between the Stats and ML-communities and mindsets. The emerging field of data science provides a key opportunity to forge a new approach for analyzing and interpreting large and complex data merging multiple fields.