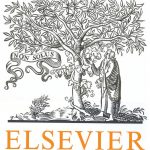
ترجمه مقاله نقش ضروری ارتباطات 6G با چشم انداز صنعت 4.0
- مبلغ: ۸۶,۰۰۰ تومان
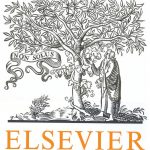
ترجمه مقاله پایداری توسعه شهری، تعدیل ساختار صنعتی و کارایی کاربری زمین
- مبلغ: ۹۱,۰۰۰ تومان
abstract
Laplacian support vector machine (LapSVM) has received much concern in semi-supervised learning (SSL) field. To further improve its computational speed, many efficient algorithms have been developed. However, they just focus on the method of solving optimization problem not the scale of the problem itself. Inspired by the sparsity of LapSVM, in this paper, an efficient safe screening rule for LapSVM (SSR-LapSVM) is proposed to address this issue. The proposed method could significantly accelerate the original LapSVM. Through the rule, most of the training samples can be eliminated before solving optimization problem without sacrificing the optimal solution. An important advantage is the safety, in the sense that the solution is exactly the same as the original LapSVM. Different from most existing methods, our approach can effectively deal with the multiple parameter problems. Experiments on both 3 artificial datasets and 24 real world benchmark datasets demonstrate its feasibility and efficiency.
5. Conclusion
In this paper, an efficient SSR for LapSVM is proposed via variational inequalities. It can greatly reduce the number of training samples and effectively improve the computational speed. Moreover the same solution can be achieved as solving the original problem, so it is safe. In comparison with the existing DVI-SVM, it effectively addresses the problem of multiple parameters. Experiments on both 3 artificial datasets and 24 real world benchmark datasets demonstrate its feasibility and validity. The SSR only screens the labeled samples, how to screen the unlabeled instances is our further work.