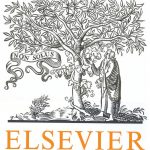
ترجمه مقاله نقش ضروری ارتباطات 6G با چشم انداز صنعت 4.0
- مبلغ: ۸۶,۰۰۰ تومان
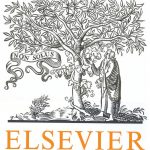
ترجمه مقاله پایداری توسعه شهری، تعدیل ساختار صنعتی و کارایی کاربری زمین
- مبلغ: ۹۱,۰۰۰ تومان
Abstract
This paper proposes a novel approach for intrusion detection system based on sampling with Least Square Support Vector Machine (LS-SVM). Decision making is performed in two stages. In the first stage, the whole dataset is divided into some predetermined arbitrary subgroups. The proposed algorithm selects representative samples from these subgroups such that the samples reflect the entire dataset. An optimum allocation scheme is developed based on the variability of the observations within the subgroups. In the second stage, least square support vector machine (LS-SVM) is applied to the extracted samples to detect intrusions. We call the proposed algorithm as optimum allocation-based least square support vector machine (OALS-SVM) for IDS. To demonstrate the effectiveness of the proposed method, the experiments are carried out on KDD 99 database which is considered a de facto benchmark for evaluating the performance of intrusions detection algorithm. All binary-classes and multiclass are tested and our proposed approach obtains a realistic performance in terms of accuracy and efficiency. Finally a way out is also shown the usability of the proposed algorithm for incremental datasets.
Conclusion
Accurate detection of various types of attack in IDS is a complicated problem, requiring the analysis of large sets of IDS data. Representative samples from a large data set play an important role to detect intrusions in the field of network security. However the current solutions for detecting intrusions is only for static datasets. This paper proposes an IDS that can be used both for static and incremental data. The proposed IDS uses the idea of sampling and we refer to this as the optimum allocation based least square support vector machine (OA-LS-SVM). The proposed methodology is discussed and validated through KDD 99 dataset which is considered as a benchmark for testing any IDS approach. The experimental results show that the proposed method is every effective for detecting intrusions for static (i.e., the entire dataset is assumed to be available at the time of release) as well as for incremental datasets.