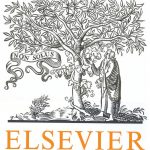
ترجمه مقاله نقش ضروری ارتباطات 6G با چشم انداز صنعت 4.0
- مبلغ: ۸۶,۰۰۰ تومان
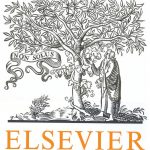
ترجمه مقاله پایداری توسعه شهری، تعدیل ساختار صنعتی و کارایی کاربری زمین
- مبلغ: ۹۱,۰۰۰ تومان
Abstract
In this paper, we propose a deep learning framework for malware classification. There has been a huge increase in the volume of malware in recent years which poses a serious security threat to financial institutions, businesses and individuals. In order to combat the proliferation of malware, new strategies are essential to quickly identify and classify malware samples so that their behavior can be analyzed. Machine learning approaches are becoming popular for classifying malware, however, most of the existing machine learning methods for malware classification use shallow learning algorithms (e.g. SVM). Recently, Convolutional Neural Networks (CNN), a deep learning approach, have shown superior performance compared to traditional learning algorithms, especially in tasks such as image classification. Motivated by this success, we propose a CNN-based architecture to classify malware samples. We convert malware binaries to grayscale images and subsequently train a CNN for classification. Experiments on two challenging malware classification datasets, Malimg and Microsoft malware, demonstrate that our method achieves better than the state-of-the-art performance. The proposed method achieves 98.52% and 99.97% accuracy on the Malimg and Microsoft datasets respectively.
CONCLUSION AND FUTURE WORK
Malware is increasingly posing a serious security threat to computer systems. It is essential to analyze the behavior of malware and categorize samples so that robust programs to prevent malware attacks can be developed. Towards this endeavor, we have proposed a deep convolutional neural network (CNN) architecture for malware classification. We first convert malware samples to grayscale images and then train a CNN for classification. Experimental results on two benchmark malware classification datasets shows the effectiveness of our proposed method.