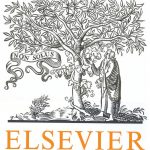
ترجمه مقاله نقش ضروری ارتباطات 6G با چشم انداز صنعت 4.0
- مبلغ: ۸۶,۰۰۰ تومان
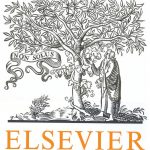
ترجمه مقاله پایداری توسعه شهری، تعدیل ساختار صنعتی و کارایی کاربری زمین
- مبلغ: ۹۱,۰۰۰ تومان
abstract
Article history: Received 15 September 2016 Received in revised form 20 December 2016 Accepted 27 December 2016 Available online 8 January 2017 The remarkable advances in biotechnology and health sciences have led to a significant production of data, such as high throughput genetic data and clinical information, generated from large Electronic Health Records (EHRs). To this end, application of machine learning and data mining methods in biosciences is presently, more than ever before, vital and indispensable in efforts to transform intelligently all available information into valuable knowledge. Diabetes mellitus (DM) is defined as a group of metabolic disorders exerting significant pressure on human health worldwide. Extensive research in all aspects of diabetes (diagnosis, etiopathophysiology, therapy, etc.) has led to the generation of huge amounts of data. The aim of the present study is to conduct a systematic review of the applications of machine learning, data mining techniques and tools in the field of diabetes research with respect to a) Prediction and Diagnosis, b) Diabetic Complications, c) Genetic Background and Environment, and e) Health Care and Management with the first category appearing to be the most popular. A wide range of machine learning algorithms were employed. In general, 85% of those used were characterized by supervised learning approaches and 15% by unsupervised ones, and more specifically, association rules. Support vector machines (SVM) arise as the most successful and widely used algorithm. Concerning the type of data, clinical datasets were mainly used. The title applications in the selected articles project the usefulness of extracting valuable knowledge leading to new hypotheses targeting deeper understanding and further investigation in DM.
7. Conclusions
In this study, a systematic effort was made to identify and review machine learning and data mining approaches applied on DM research. DM is rapidly emerging as one of the greatest global health challenges of the 21st century. To date, there is a significant work carried out in almost all aspects of DM research and especially biomarker identification and prediction-diagnosis. The advent of biotechnology, with the vast amount of data produced, along with the increasing amount of EHRs is expected to give rise to further in-depth exploration toward diagnosis, etiopathophysiology and treatment of DM through employment of machine learning and data mining techniques in enriched datasets that include clinical and biological information.