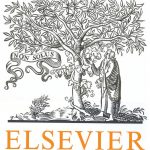
ترجمه مقاله نقش ضروری ارتباطات 6G با چشم انداز صنعت 4.0
- مبلغ: ۸۶,۰۰۰ تومان
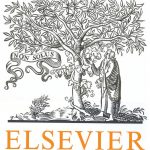
ترجمه مقاله پایداری توسعه شهری، تعدیل ساختار صنعتی و کارایی کاربری زمین
- مبلغ: ۹۱,۰۰۰ تومان
Abstract
Intrusion Detection Systems (IDS) are used in computer networks to safeguard the integrity and confidentiality of sensitive data. In recent years, network traffic has become sizeable enough to be considered under the big data domain. Current machine learning based techniques used in IDS are largely defined on eager learning paradigms which lose performance efficiency by trying to generalize training data before receiving queries thereby incurring overheads for trivial computations. This paper, proposes the use of lazy learning methodologies to improve overall performance of IDS. A novel heuristic weight based indexing technique has been used to overcome the drawback of high search complexity inherent in lazy learning. IBk and LWL, two popular lazy learning algorithms have been compared and applied on the NSL-KDD dataset for simulating a real-world like scenario and comparing their relative performances with hw-IBk. The results of this paper clearly indicate lazy algorithms as a viable solution for real-world network intrusion detection.
Conclusion
This paper elucidates the advantages of lazy learning in IDS. Lazy learning improves the efficiency of the NIDS by eliminating pre-fetching of overheads that are inherent in eager learning algorithms popularly in use today. Further an improvement of the k-nearest neighbour algorithm has been proposed to reduce the search complexity using a heuristic weight based indexing system. The results of this sufficiently prove thehw-IBk algorithm is a practical and viable solution for intrusion detection in data streams, with great accuracy,more so than other machine learning algorithms currently deployed. Additionally, the IBk algorithm has been compared to another other lazy learning algorithmLWL in order to compareand contrast their performances on the NSL-KDD network traffic dataset.The time taken to detect intrusions is significantly reduced and it is observed that the number of correctly classified instances of intrusions is relatively higher (~97.59).Thus, with significant increase in the speed of computation, network intrusions can now be detected faster without any loss to accuracy and thus aid in threat identification in real-time network system.