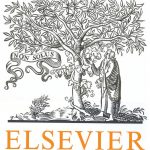
ترجمه مقاله نقش ضروری ارتباطات 6G با چشم انداز صنعت 4.0
- مبلغ: ۸۶,۰۰۰ تومان
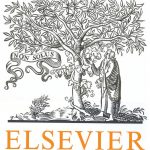
ترجمه مقاله پایداری توسعه شهری، تعدیل ساختار صنعتی و کارایی کاربری زمین
- مبلغ: ۹۱,۰۰۰ تومان
Abstract
Many real-world data can be represented as attributed graphs that contain vertices each of which is associated with a set of attribute values. Discovering clusters, or communities, which are structural patterns in these graphs is one of the most important tasks in graph analysis. To perform the task, a number of algorithms have been proposed. Some of them detect clusters of particular topological properties whereas some others discover them based mainly on attribute information. Also, most algorithms discover disjoint clusters only. As a result, they may not be able to detect more meaningful clusters hidden in the attributed graph. To do so more effectively, we propose an algorithm, called FSPGA, to discover fuzzy structural patterns for graph analytics. FSPGA performs the task of clusters discovery as a fuzzy constrained optimization problem which takes into consideration both graph topology and attribute values. FSPGA has been tested with both synthetic and real-world graph data sets and is found to be efficient and effective at detecting clusters in attributed graphs. FSPGA is a promising fuzzy algorithm for structural pattern detection in attributed graphs.
V. CONCLUSION
In this paper, FSPGA, which is an algorithm for discovering fuzzy structural patterns in the form of clusters in the attributed graph, is proposed. Compared with prevalent algorithms that take different properties of an attributed graph, including topology, attribute, and both of the aforementioned, FSPGA may find an optimal arrangement of clusters for vertices in an attributed graph by formulating the task as a fuzzy constrained optimization problem. As the adoption of fuzzy set theory when determining the cluster membership, FSPGA can detect overlapping clusters, while most of the prevalent algorithms cannot. The experimental results presented in this paper show that FSPGA may perform robustly and efficiently in different types graph data, compared with the classical, latest graph clustering algorithms, and fuzzy clustering algorithms. In future, we will intend to further improve the efficiency of FSPGA and develop a version of FSPGA that may discover hierarchical structural patterns in attributed graphs.