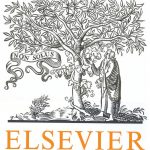
ترجمه مقاله نقش ضروری ارتباطات 6G با چشم انداز صنعت 4.0
- مبلغ: ۸۶,۰۰۰ تومان
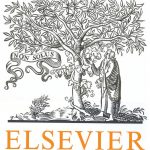
ترجمه مقاله پایداری توسعه شهری، تعدیل ساختار صنعتی و کارایی کاربری زمین
- مبلغ: ۹۱,۰۰۰ تومان
ABSTRACT
Polyadenylation (Poly(A)) plays crucial roles in gene regulation, especially in messenger RNA metabolism, protein diversification and protein localization. Accurate prediction of polyadenylation sites and identification of motifs that controlling polyadenylation are fundamental for interpreting the patterns of gene expression, improving the accuracy of genome annotation and comprehending the mechanisms that governing gene regulation. Despite considerable advances in using machine learning techniques for this problem, its efficiency is still limited by the lack of experiences and domain knowledge to carefully design and generate useful features, especially for plants. With the increasing availability of extensive genomic datasets and leading computational techniques, deep learning methods, especially convolutional neural networks, have been applied to automatically identify and understand gene regulation directly from gene sequences and predict unknown sequence profiles. Here, we present DeepPolyA, a new deep convolutional neural network-based approach, to predict polyadenylation sites from the plant Arabidopsis thaliana gene sequences. We investigate various deep neural network architectures and evaluate their performance against classical machine learning algorithms and several popular deep learning models. Experimental results demonstrate that DeepPolyA is substantially better than competing methods regarding various performance metrics. We further visualize the learned motifs of DeepPolyA to provide insights of our model and learned polyadenylation signals.
CONCLUSION AND FUTURE WORKS
In this paper, we proposed DeepPolyA, a deep convolutional neural network approach, to automatically and accurately modeling the poly(A) signals. Using the plant Arabidopsis thaliana gene sequences datasets with one-hot encoding method, we trained several competing deep learning models with various architectures and compared the classification performance with baseline machine learning methods through several significant metrics. The evaluation results show that DeepPolyA outperforms all the competing methods without involving extensive manual feature engineering. We visualized the learned motifs of the first convolutional layer using TOMTOM against the JASPAR motif datasets to demonstrate that DeepPolyA can automatically extract poly(A) signals and features from the raw sequence data.