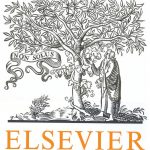
ترجمه مقاله نقش ضروری ارتباطات 6G با چشم انداز صنعت 4.0
- مبلغ: ۸۶,۰۰۰ تومان
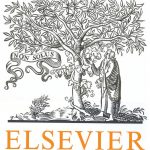
ترجمه مقاله پایداری توسعه شهری، تعدیل ساختار صنعتی و کارایی کاربری زمین
- مبلغ: ۹۱,۰۰۰ تومان
ABSTRACT
An encephalogram (EEG) is a commonly used ancillary test to aide in the diagnosis of epilepsy. The EEG signal contains information about the electrical activity of the brain. Traditionally, neurologists employ direct visual inspection to identify epileptiform abnormalities. This technique can be time-consuming, limited by technical artifact, provides variable results secondary to reader expertise level, and is limited in identifying abnormalities. Therefore, it is essential to develop a computer-aided diagnosis (CAD) system to automatically distinguish the class of these EEG signals using machine learning techniques. This is the first study to employ the convolutional neural network (CNN) for analysis of EEG signals. In this work, a 13-layer deep convolutional neural network (CNN) algorithm is implemented to detect normal, preictal, and seizure classes. The proposed technique achieved an accuracy, specificity, and sensitivity of 88.67%, 90.00% and 95.00%, respectively.
CONCLUSION
A novelty of this proposed model is being the first application of deep neural network for EEG-based seizure detection. A 13-layer deep learning CNN algorithm is implemented for the automated EEG analysis. An average accuracy of 88.7% is obtained with a specificity of 90% and a sensitivity of 95%. The performance (accuracy, sensitivity, and specificity) of proposed model is slightly lower than some of the works summarized in Table 4. The advantage of the model presented in this paper, however, is separate steps of feature extraction and feature selection are not required in this work. Nevertheless, the main drawback of this work is the lack of huge EEG database. Proposed algorithm requires a diversity of data to obtain an optimum performance. The performance of this technique can be improved by applying a bagging algorithm and increasing the number of samples.