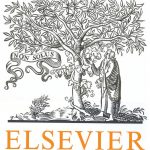
ترجمه مقاله نقش ضروری ارتباطات 6G با چشم انداز صنعت 4.0
- مبلغ: ۸۶,۰۰۰ تومان
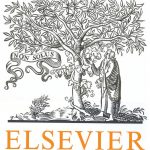
ترجمه مقاله پایداری توسعه شهری، تعدیل ساختار صنعتی و کارایی کاربری زمین
- مبلغ: ۹۱,۰۰۰ تومان
Abstract
In recent years, learning management systems (LMSs) have played a fundamental role in higher education teaching models. A new line of research has been opened relating to the analysis of student behavior within an LMS, in the search for patterns that improve the learning process. Current e-learning platforms allow for recording student activity, thereby enabling the exploration of events generated in the use of LMS tools. This paper presents a case study conducted at the Catholic University of Murcia, where student behavior in the past four academic years was analyzed according to learning modality (that is, oncampus, online, and blended), considering the number of accesses to the LMS, tools employed by students and their associated events. Given the difficulty of managing the large volume of data generated by users in the LMS (up to 70 GB in this study), statistical and association rule techniques were performed using a Big Data framework, thus speeding up the statistical analysis of the data. The obtained results are demonstrated using visual analytic techniques, and evaluated in order to detect trends and deficiencies in the use of the LMS by students.
Conclusion and future work
The main goal of this paper resided in obtaining knowledge from data stored 545 in an e-learning platform, such as the Sakai LMS. We have proposed the use of big data technologies and a framework to attempt to obtain student behavior patterns and be able to provide conclusions to increase student performance by improving their learning process. We selected a big data solution based on Azure HDInsight using its HDFS implementation. The tool used to transfer 550 data from Sakai database was Sqoop, and these data were stored in a Hive [22] data warehouse. Moreover, we implemented the Apriori algorithm following the Hadoop MapReduce framework in order to obtain association rules for the events performed by students in the Sakai LMS. Using these technologies and the big data framework, we studied and analyzed a database containing 70 GB 555 of information regarding the behavior of UCAM students, including all of the available data on degrees and masters. The obtained results have been discussed, translated, and visually depicted visually in order to be easily interpreted by people who are not related to the big data field, such as degree coordinators, lecturers or students.