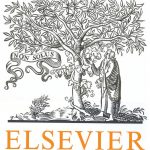
ترجمه مقاله نقش ضروری ارتباطات 6G با چشم انداز صنعت 4.0
- مبلغ: ۸۶,۰۰۰ تومان
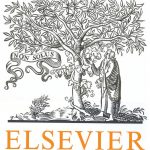
ترجمه مقاله پایداری توسعه شهری، تعدیل ساختار صنعتی و کارایی کاربری زمین
- مبلغ: ۹۱,۰۰۰ تومان
This article provides a survey of recent results on exploiting parametric auto-regressive (AR) models for adaptive radar target detection. Specifically, three types of radar systems are considered, including phased-array radar with multiple co-located transmitters and receivers, distributed multi-input multi-output (MIMO) radar with widely and spatially separated transmitters and receivers, and passive radar which uses existing sources as illuminators of opportunity (IOs). These radar systems are of significant interest for a wide range of military and civilian applications. For each of the three types of radars, we discuss how AR processes can be employed to succinctly model the underlying signal correlation and efficiently estimate it from limited data, thus enabling effective target detection in complex non-homogeneous environments when training data is limited. We illustrate the performance of such parametric model assisted detectors relative to conventional non-parametric approaches by using computer simulated and experimental data.
I. INTRODUCTION
Parametric modeling is frequently used in the design and analysis of radar systems (e.g., [1]–[10]). It employs physical or statistical models consisting of a finite number of parameters that are useful in the representation and processing of a signal for radar functions. There are many parametric models available for radar applications, e.g., geometrical theory of diffraction model [1], hidden Markov model [2], compound Gaussian model [3], and auto-regressive (AR) model [4]–[10], etc. The AR model has attracted extensive research efforts for radar target detection since it can fit both Gaussian and non-Gaussian observations. In addition, parameter estimation of the AR model is relatively simple, which usually involves solving a system of linear equations.
V. CONCLUSION
In this survey article, we have shown that AR processes can be employed to model the clutter and unknown source signal to reduce the requirement of training data for effective target detection. There are some subtle differences in applying parametric modeling in solving different radar detection problems. Specifically, in phased-array radar, the disturbance is modeled as multi-channel AR process. For distributed MIMO radar, a set of independent scalar AR processes are used to model the clutter observed at different Tx-Rx pairs, which are capable of capturing the variations in both clutter structure and power level across different probing-observing angles. Finally, for passive radar, the unknown IO signal is treated as an element-correlated stochastic process, which can be represented by an AR model. In all cases, we have demonstrated that AR modeling is able to bring significant performance improvement over conventional non-parametric methods, in particular when training data is limited.
این مقاله به بررسی نتایج اخیر در زمینه استفاده از مدل های پارامتری خود همبسته برای تشخیص تطبیقی هدف رادار می پردازد. به بیان دقیق تر، سه نوع سامانه راداری بررسی می گردد که شامل رادار آرایه فازی با چندین گیرنده و فرستنده هم مکان، رادار چند ورودی چند خروجی توزیع شده (MIMO) با گیرنده ها و فرستنده های گسترده و مکانمند و رادار غیر فعال می شوند. رادارهای غیر فعال با نام فرستنده های مغتنم (IO ها) شناخته می شوند که از منابع موجود بهره می برند. این رادارها در بسیاری از زمینه های نظامی و غیر نظامی کاربرد دارند. در هر سه مورد رادار بیان شده، ما به بررسی این موضوع می پردازیم که چگونه فرآیندهای AR را می توان برای مدل سازی دقیق همبستگی سیگنال بنیادی و برآورد کارآمد آن از داده های محدود به کار برد. با این کار، تشخیص موثر هدف در محیط های پیچیده ناهمگون، در زمانی که محدودیت داده وجود دارد، امکان پذیر می شود. با استفاده از داده های شبیه سازی شده و آزمایشی، عملکرد مدل پارامتری مورد نظر با کمک آشکارگرها نسبت به رویکردهای غیر پارامتری سنتی شرح داده خواهد شد.
I. مقدمه
مدل سازی پارامتری در طراحی و تحلیل سامانه های راداری به طور گسترده مورد استفاده قرار گرفته است (مانند (1)-(10)). این مورد از مدل های فیزیکی یا آماری متشکل از چند پارامتر محدود استفاده می کند که در نمایش و پردازش سیگنال توابع رادار کاربرد دارند. از سوی دیگر، مدل های پارامتری مختلفی برای برنامه های راداری وجود دارد مانند نظریه هندسی مدل پراش (1)، مدل مارکوف مخفی (2)، مدل ترکیب گاوسی (3) و مدل خود هبستگی (AR) (4)-(10) و غیره. مدل AR اقدامات پژوهشی گسترده را در زمینه تشخیص هدف رادار به خود جلب کرده است زیرا هم متناسب با مشاهدات گاوسی و هم غیر گاوسی می باشد. علاوه بر این، برآورد پارامتری مدل AR نسبتا ساده است که معمولا شامل حل معادلات خطی سامانه می شود.
V. نتیجه گیری
در این مقاله پژوهشی، ما نشان دادیم که فرآیندهای AR را می توان برای مدل سازی آشوب و سیگنال منبع نامعلوم به کار برد و نیاز به داده های آموزشی برای تشخیص موثر هدف را کاهش داد. تفاوت های مختصری در بکارگیری مدل سازی پارامتری در حل مسائل مختلف تشخیص رادار وجود دارد. به بیان دقیق تر، در رادار آرایه فازی، اختلال به عنوان یک فرآیند AR چند کاناله مدل سازی می شود. در رادار MIMO توزیع شده، مجموعه ای از فرآیندهای AR مقیاس پذیر مستقل برای مدل سازی آشوب مشاهده شده در جفت های مختلف Tx-Rx مورد استفاده قرار می گیرند که قادرند نوسانات را در ساختار آشوب و سطح توان بین زوایای کاوش- مشاهده مختلف ثبت کنند. در نهایت، در رادار غیر فعال، سیگنال IO نامعلوم به عنوان یک فرآیند احتمالی با عضو همبسته در نظر گرفته می شود که می توان آن را با مدل AR ارائه کرد. در تمام موارد، ما نشان داده ایم که مدل سازی AR می تواند بهبود عملکرد قابل ملاحظه ای را در مقایسه با روش های غیر پارامتری سنتی و علی الخصوص زمانی که محدودیت در داده های آموزشی وجود دارد، ارائه کند.