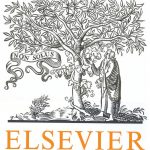
ترجمه مقاله نقش ضروری ارتباطات 6G با چشم انداز صنعت 4.0
- مبلغ: ۸۶,۰۰۰ تومان
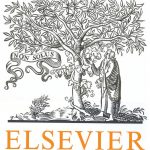
ترجمه مقاله پایداری توسعه شهری، تعدیل ساختار صنعتی و کارایی کاربری زمین
- مبلغ: ۹۱,۰۰۰ تومان
A huge amount of data is being collected and stored by financial institutions like banks during their operations. These data contain the most important facts about the institutions and its customers. A good and efficient data analytics system can find patterns in this huge data source that can be used in actionable knowledge creation. Actionable knowledge is the knowledge that can be put to decision making and take some positive action towards better performance of organizations. This actionable knowledge is termed Business Intelligence by data scientists. Business Intelligence and Analytics is the process of applying data mining techniques to organizational or corporate data to discover patterns. Business Intelligence and Business Analytics are emerging as important and essential fields both for data scientists and organizations. Risk analysis, fraud detection, customer retention, customer satisfaction analysis and actuarial analysis are some of the areas of application of business intelligence and analytics. Credit risk analysis is an important part of a successful financial institution particularly in the banking sector. The current study takes this risk analysis in financial institutions and reviews the state of the art in using data analytics or data mining techniques for financial risk analysis. The analysis of risk from financial data depends on several factors that are both objective and subjective. Hence it is a multi-criteria decision problem. The study also proposes a multi-objective genetic algorithm (MOGA) for analyzing financial data for risk analysis and prediction. The proposed MOGA is different from other evolutionary systems in that a memory component to hold the rules is added to the system while other systems in the literature are memory less. The algorithm is applied to bench mark data sets for predicting the decision on credit card and credit applications. The preliminary results are encouraging and show light towards better decision making in reducing risks.
1 Introduction
Organizations form the back bone of every country siphoning money out and into the system. Financial institutions are organizations that provide services including lending money to individual persons as well as to huge organizations. Therefore they are directly involved in the financial strength of a country. All types of organization whether product or service have to deal with several risks arising due to a variety of reasons. Financial institutions are prone to more risks and they cannot operate without taking risks. Risk causes a great deal of potential damage and inconvenience for the enterprise stakeholders (Wu and Birge 2016). Thus organizations need to use different strategies to manage or avoid risks. Therefore it becomes important for financial institutions to model risks using historical data in order to gain insight into the risk patterns, so that it falls under their acceptable thresholds.
6 Conclusion and Future Work
The study undertaken has considered risk assessment in financial institutions as a multi-criteria decision making problem. Since evolutionary systems are best at dealing with multi-criteria and multi-objective problems, a genetic algorithm is proposed and applied on credit card application and Australian credit approval data sets which are bench mark data sets from the UCI machine learning repository concerning credit risks. The results are encouraging in that the algorithm discovers valid patterns from the data. Moreover the algorithm is able to create a large set of unique rules and best rules. However, the algorithm uses threshold values for the evaluation metrics to choose rules for the next generation rather than an optimization strategy like Pareto optimization. Therefore as future work we propose to use a Pareto optimization strategy to improve both the convergence of the solution and to improve the classification accuracy and decrease the complexity of the classifier. The study also proposes to use other rule evaluation metrics like interestingness of the rules to observe their influence on the convergence of solutions and accuracy.