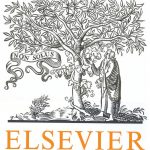
ترجمه مقاله نقش ضروری ارتباطات 6G با چشم انداز صنعت 4.0
- مبلغ: ۸۶,۰۰۰ تومان
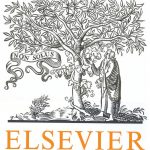
ترجمه مقاله پایداری توسعه شهری، تعدیل ساختار صنعتی و کارایی کاربری زمین
- مبلغ: ۹۱,۰۰۰ تومان
Abstract
This paper presents a novel technique of finding a convex combination of outputs of anomaly detectors maximizing the accuracy in τ-quantile of most anomalous samples. Such an approach better reflects the needs in the security domain in which subsequent analysis of alarms is costly and can be done only on a small number of alarms. An extensive experimental evaluation and comparison to prior art on real network data using sets of anomaly detectors of two existing intrusion detection systems shows that the proposed method not only outperforms prior art, it is also more robust to noise in training data labels, which is another important feature for deployment in practice.
5. Conclusion
This paper has proposed a new algorithm for finding a convex combination of anomaly detectors maximizing accuracy at τ - quantile of returned samples, which is a scenario frequently appearing in the security field. The algorithm assumes labeled data, which are difficult to obtain and rarely perfect in security domains. Therefore, an emphasis was put on the experimental study, involving two different types of intrusion detection systems, eight types of combination functions, 34 different network captures containing more than 20 million of samples of behavior of different algorithms under different types of noise. The experimental results show that the proposed method is more accurate than prior art in finding a good combination of detectors with high accuracy in returned samples. The results also show that supervised methods can easily overfit if some type of malicious behavior is completely missing in the training data or is incorrectly labeled (mistake of labeling oracle). The severity of the overfitting depends on how much different types of malicious behavior are similar to each other. The comparison of unsupervised combination functions did not have a clear winner, since in one experimental setting mean rank was the best while in the second one it was mean. The presented experimental results show that future efforts should be directed toward finding methods combining good properties of both supervised and unsupervised combination functions.