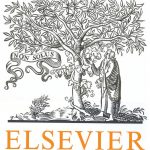
ترجمه مقاله نقش ضروری ارتباطات 6G با چشم انداز صنعت 4.0
- مبلغ: ۸۶,۰۰۰ تومان
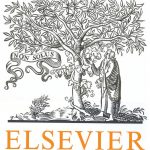
ترجمه مقاله پایداری توسعه شهری، تعدیل ساختار صنعتی و کارایی کاربری زمین
- مبلغ: ۹۱,۰۰۰ تومان
The fact that semantics must play a crucial role in the sentiment interpretation of text is rather obvious, as even just considering the plain meaning of words can be very indicative (“I liked it” vs. “I hated it”). However, things are not that simple or straightforward for at least two reasons: (1) meaning is not so easy to define, detect, and extract automatically, and (2) sentiment analysis is often not just a matter of distinguishing positive from negative opinions, especially in recent developments.
In the 2015 SemEval campaign, four shared tasks were organized within the Sentiment Analysis track: a rather general task on sentiment analysis in Twitter (task 10 [1], with four subtasks), a task focused on figurative language, entitled “Sentiment Analysis of Figurative Language in Twitter” (task 11 [2]), an aspect-based sentiment analysis task (task 12 [3]), where systems had to identify aspects of entities and the sentiment expressed for each aspect, and a rather different task focused on events’ polarity, entitled “CLIPEval Implicit Polarity of Events” (task 9 [4]). Within the ongoing SemEval 2016, there is also a task on detecting stance in tweets (task 61)—that is, detecting the position of the author with respect to a given target (against/in favor/neutral)—and one on determining sentiment intensity (task 72). Some of these tasks provide datasets in more than one language. Additionally, a shared task on concept-level sentiment analysis has been organized recently in the context of the European Semantic Web Conference [5]. This fervent, current action on stimulating research, resources, and tools in this field by organizing more numerous and more complex tasks tells us not only that interest in sentiment analysis is growing but also that sentiment analysis is no longer just about detecting whether a given review or tweet is objective or subjective, and in the latter case it is whether positive or negative. Rather, it requires a more complex analysis and interpretation of messages that in turn must rely on deeper processing and understanding. Thus although it is true that semantics and semantic processing play a crucial role in this, we must see how this happens, from several points of view.
4 CONCLUSIONS
We started this contribution by pointing out how much interest and research in sentiment analysis have grown in the past few years. We have highlighted that in the recent SemEval campaigns tasks more complex than basic polarity classification have been proposed. And complexity here means finergrained interpretations of sentiment, be it related to figurative uses such as irony or be it related to singling out which entities or entity properties are actually in focus in an opinionated statement. This is also reflected in resource creation, where we have seen that corpora have been extended in their annotation to include polarity at the sentence level, where before this was done at the global document level, or at the entity level, where before it was done at the message level.