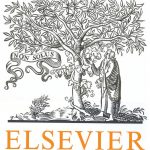
ترجمه مقاله نقش ضروری ارتباطات 6G با چشم انداز صنعت 4.0
- مبلغ: ۸۶,۰۰۰ تومان
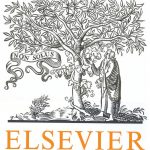
ترجمه مقاله پایداری توسعه شهری، تعدیل ساختار صنعتی و کارایی کاربری زمین
- مبلغ: ۹۱,۰۰۰ تومان
Abstract
A mathematical model for variable selection in functional linear regression models with scalar response is proposed. By “variable selection” we mean a procedure to replace the whole trajectories of the functional explanatory variables with their values at a finite number of carefully selected instants (or “impact points”). The basic idea of our approach is to use the Reproducing Kernel Hilbert Space (RKHS) associated with the underlying process, instead of the more usual L 2 [0, 1] space, in the definition of the linear model. This turns out to be especially suitable for variable selection purposes, since the finite-dimensional linear model based on the selected “impact points” can be seen as a particular case of the RKHS-based linear functional model. In this framework, we address the consistent estimation of the optimal design of impact points and we check, via simulations and real data examples, the performance of the proposed method.
7. Conclusions
The RKHS approach we have introduced in this paper provides a natural framework for a formal unified theory of variable selection for functional data. The “sparse” models (those where the variable selection techniques are fully justified) appear as particular cases in this setup. As a consequence, it is possible to derive asymptotic consistency results as those obtained in the paper. Likewise, it is also possible to consider the problem of estimating the “true” number of relevant variables in a consistent way, as we do in Section 4. This is in contrast with other standard proposals for which the number of variables is previously fixed as an input, or it is determined using cross validation and other computationally expensive methods. Then, our proposal is more firmly founded in theory and, at the same time, provides a much faster method in practice, which is important when dealing with large data sets.
The empirical results we have obtained are encouraging. In short, according to our experiments, the RKHS-based method works better than other variable selection methods is those sparse models that fulfill the ideal theoretical conditions we need. In the non sparse model considered in the simulations, the RKHS method is slightly outperformed by other proposals (but still behaves reasonably). Finally, in the “neutral” field of real data examples the performance looks also satisfactory and competitive.
Last but not least, from a general, methodological point of view, this paper represents an additional example of the surprising usefulness of reproducing kernels in statistics. Additional examples can be found in [3, 5, 17, 21, 32].