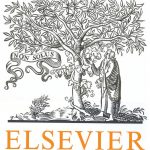
ترجمه مقاله نقش ضروری ارتباطات 6G با چشم انداز صنعت 4.0
- مبلغ: ۸۶,۰۰۰ تومان
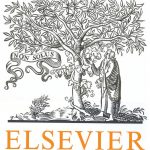
ترجمه مقاله پایداری توسعه شهری، تعدیل ساختار صنعتی و کارایی کاربری زمین
- مبلغ: ۹۱,۰۰۰ تومان
abstract
Recent theoretical work suggests that signs of asset returns are predictable given that their volatilities are. This paper investigates this conjecture using information demand, approximated by the daily internet search volume index (SVI) from Google. Our results reveal that incorporating the SVI variable in various GARCH family models significantly improves volatility forecasts. Moreover, we demonstrate that the sign of stock returns is predictable contrary to the levels, where predictability has proven elusive in the US context. Finally, we provide novel evidence on the economic value of sign predictability and show that investors can form profitable investment strategies using the SVI.
5. Conclusion
In this paper we empirically investigate whether a measure for information demand, can lead to better sign forecasts of stock returns. This is of particular interest given that US stock returns have been proven difficult to predict in the levels. Specifically, we augment a number of competing GARCH family models with a measure of information demand (approximated by SVI from Google) and construct one-step-ahead volatility forecasts, which we use within the Christoffersen and Diebold (2006) framework to predict the sign of stock returns. We then focus on the economic significance of sign predictability and formulate appropriate trading strategies from the perspective of a real-time investor. This is a very important dimension of our study given the lack of empirical evidence within this framework and the fact that improved sign predictability in a statistical sense does not necessarily mean higher economic value for investors. In particular, we adopt the approach by Granger and Pesaran (2000) and consider an investor whose goal is to maximize her utility function using a time-varying optimal rule which translates sign probability forecasts into investment decisions. Our empirical application relies on daily data from the S&P 500 index and covers the period from January 2, 2004 through December 31, 2016.
Turning to our results, we show that extending all considered GARCH family models with the change in demand for information variable (DSVI) leads to more accurate return volatility forecasts. We find that this improvement in forecasting power is statistically significant and robust across different sample periods (including the recent financial crisis). This result is in line with a small body of relevant work and highlights the usefulness of the DSVI in predicting the volatility of returns during both normal and turbulent periods in the stock market.