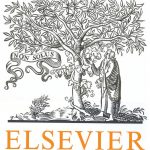
ترجمه مقاله نقش ضروری ارتباطات 6G با چشم انداز صنعت 4.0
- مبلغ: ۸۶,۰۰۰ تومان
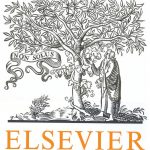
ترجمه مقاله پایداری توسعه شهری، تعدیل ساختار صنعتی و کارایی کاربری زمین
- مبلغ: ۹۱,۰۰۰ تومان
Online social networks have become popular platforms for spammers to spread malicious content and links. Existing state-of-the-art optimization methods mainly use one kind of user-generated information (i.e., single view) to learn a classification model for identifying spammers. Due to the diversity and variability of spammers' strategies, spammers' behavior may not be completely characterized only by single view's information. To tackle this challenge, we first statistically analyze the importance of considering multiple view information for spammer detection task on a large real-world Twitter dataset. Accordingly, we propose a generalized social spammer detection framework by jointly integrating multiple view information and a novel social regularization term into a classification model. To keep the completeness of the original dataset and detect more spammers by the proposed method, we introduce a simple strategy to fill the missing data for each view. Experimental results on a real-world Twitter dataset show that the proposed method outperforms the existing methods significantly.
1. Introduction
Online social networks (OSNs), such as Twitter and Facebook, have become popular platforms to disseminate and share information [1]. Unfortunately, social spammers take advantage of those platforms to spread phishing scams, publish malicious content and links, and promote commodity information [2–4]. According to a study by Nexgate [5], the number of social spam grew more than 355% from January to July of 2013, which means that one in two hundred social messages was a spam, and 15% of all spams contained URLs linking to risky websites. Spammers are so sophisticated and concealed that they change spamming strategies irregularly and try to disguise as legitimate users. Moreover, to increase their influence and be undetected, spammers collude with each other to construct the criminal communities [6]. The malicious behavior of spammers has not only hindered the OSNs' development largely [7], but also threatened information security and personal privacy [8]. Therefore, it is crucial to design effective and novel spammer detection methods for the development of social systems.
7. Conclusion
In this paper, we propose a generalized framework by taking advantage of multiple views' information for social spammer detection. Different from the existing methods that utilize single view's information, the proposed method MVSD integrates multiple social information based on NMF with classification model into a learning model. Moreover, we introduce a simple strategy to complement the missing values of different view to predict more users, thus improving the performance of detection spammers. Experimental results on a real dataset show that MVSD obtains the better detection performance than the existing methods even with a small number of training data.