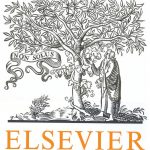
ترجمه مقاله نقش ضروری ارتباطات 6G با چشم انداز صنعت 4.0
- مبلغ: ۸۶,۰۰۰ تومان
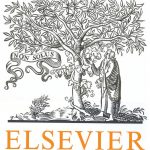
ترجمه مقاله پایداری توسعه شهری، تعدیل ساختار صنعتی و کارایی کاربری زمین
- مبلغ: ۹۱,۰۰۰ تومان
چكيده
1- مقدمه
2- پيش زمينه
2.1 امتياز دهيِ اعتباري
2.2 الگوريتم هاي انتخاب ويژگي
2.3 الگوريتم هاي طبقه بنديِ مبنا(مستقل)
2.4: الگوريتم هاي طبقه بندي گروهي
3- طرح آزمايش
3.1: تشريح مجموعه داده ها و پيش پردازشِ آن ها
3.2 ارزيابي عملكرد
3.3 مدل پيشنهادي
4- نتايج و بحث و گفتگو
4.1 الگوريتم ژنتيكي و تعيين پارامترها
4.2 تعيين پارامترها و روش Relief
4.3 روش معیار ضریب بهره اطلاعات و تعيين پارامترها
4.4 تحليل مولفه اساسي و تعيين پارامترها
5- نتيجه گيري و پيشنهادات
چكيده
تكنيك هاي داده كاوي در حوزه بانكداري،از كاربردهاي متنوعي جهت امتياز دهيِ اعتباري برخوردار اند. يكي از معروف ترين تكنيك هاي داده كاوي،روش طبقه بندي نام دارد.مطالعات پيشين نشان دادند استفاده از الگوريتم هاي انتخاب ويژگي ها و طبقه بندي كننده هاي گروهي سبب بهبود عملكرد بانك ها در مشكلات امتياز دهيِ اعتباري مي گردد.در اين زمينه،موضوع اساسي،شبيه سازي و استفاده تركيبي از اين دو الگوريتم فوق جهت تعيين پارامترها و دستيابي به مدلي با عملكرد بالا،است . اين مقاله به نحوه استفاده از مدل تركيبيِ داده كاويِ الگوريتم هاي انتخاب ويژگي و طبقه بندي كننده هاي يادگيريِ گروهي در امتياز دهيِ اعتباري در 3 مرحله مي پردازد :1)جمع آوري و پردازش داده ها 2)اجراء 4 الگوريتمFS همچون آناليز مولفه هاي اساسي PCA،الگوريتم ژنتيكGA، معیار ضریب بهره اطلاعات و تابع ارزيابيِ صفات مهم.در اين مرحله،پارامترهاي روش FS با كمك الگوريتمِ طبقه بندي كننده ماشين بردار پشتيبان،به دقت تعيين مي شوند. پس از انتخاب مدلِ دقيق در هر ويژگي،بايد از آن ها در الگوريتم هاي طبقه بندي كننده و مبناء استفاده نمود. در مرحله فوق،بهترينِ الگوريتمFS همراه با پارامترهاي تعيين شده اش جهت استفاده در مرحلهِ مدلسازي،استفاده خواهند شد.3)اجراء الگوريتم هاي طبقه بندي بر روي مجموعه داده هاي آماده شده براي هر الگوريتمFS.نتايج مرحله 2،نشان مي دهد كه الگوريتم PCA نسبت به FS از عملكردِ مطلوب تري برخوردار است نتايج طبقه بندي مرحله 3 نيز نشان مي دهد روش آدابوست در شبكه عصبي مصنوعي(ANN) از دقت طبقه بندي بسيار بالايي برخوردار است . در نهايت،مقاله حاضر به بررسيِ مدل تركيبي به عنوان مدل عملياتي و قدرتمندي جهت اجراء امتياز دهيِ اعتباري اشاره خواهد داشت.
Abstract
Data mining techniques have numerous applications in credit scoring of customers in the banking field. One of the most popular data mining techniques is the classification method. Previous researches have demonstrated that using the feature selection (FS) algorithms and ensemble classifiers can improve the banks' performance in credit scoring problems. In this domain, the main issue is the simultaneous and the hybrid utilization of several FS and ensemble learning classification algorithms with respect to their parameters setting, in order to achieve a higher performance in the proposed model. As a result, the present paper has developed a hybrid data mining model of feature selection and ensemble learning classification algorithms on the basis of three stages. The first stage, as expected, deals with the data gathering and pre-processing. In the second stage, four FS algorithms are employed, including principal component analysis (PCA), genetic algorithm (GA), information gain ratio, and relief attribute evaluation function. In here, parameters setting of FS methods is based on the classification accuracy resulted from the implementation of the support vector machine (SVM) classification algorithm. After choosing the appropriate model for each selected feature, they are applied to the base and ensemble classification algorithms. In this stage, the best FS algorithm with its parameters setting is indicated for the modeling stage of the proposed model. In the third stage, the classification algorithms are employed for the dataset prepared from each FS algorithm. The results exhibited that in the second stage, PCA algorithm is the best FS algorithm. In the third stage, the classification results showed that the artificial neural network (ANN) adaptive boosting (AdaBoost) method has higher classification accuracy. Ultimately, the paper verified and proposed the hybrid model as an operative and strong model for performing credit scoring.