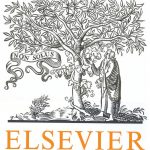
ترجمه مقاله نقش ضروری ارتباطات 6G با چشم انداز صنعت 4.0
- مبلغ: ۸۶,۰۰۰ تومان
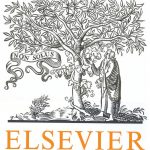
ترجمه مقاله پایداری توسعه شهری، تعدیل ساختار صنعتی و کارایی کاربری زمین
- مبلغ: ۹۱,۰۰۰ تومان
Abstract
Economists often use matched samples, especially when dealing with earnings data where a number of missing observations need to be imputed. In this paper, we demonstrate that the ordinary least squares estimator of the linear regression model using matched samples is inconsistent and has a non-standard convergence rate to its probability limit. If only a few variables are used to impute the missing data, then it is possible to correct for the bias. We propose two semiparametric bias-corrected estimators and explore their asymptotic properties. The estimators have an indirect-inference interpretation, and they attain the parametric convergence rate when the number of matching variables is no greater than four. Monte Carlo simulations confirm that the bias correction works very well in such cases.
6 Conclusion
Regression estimation using samples constructed via the NNM from two sources is not uncommon in applied economics. This paper has demonstrated that such OLS 30 estimators are generally inconsistent, and thus an appropriate bias correction is required. It has also been shown that the convergence rate to the probability limit of the OLS depends on the number of matching variables and the divergence pattern of two sample sizes.
Two versions of bias-corrected estimators have been proposed, and each can be interpreted as a variant of indirect inference estimators. The MSII estimator attains the parametric convergence rate for the cases with at most two matching variables, whereas the MSII-FM estimator achieves the parametric convergence rate when the number of matching variables does not exceed four. Monte Carlo results suggest that a small number of matches work well in practice, and in particular, we should consider the single match when the number of matching variables is two or three.
The paper aims at providing corrections for an established practice, which is to run (parametric) OLS ignoring imputation. In particular, our proposal for MSII-FM is based on a nonparametric series estimation of g2(Z) = E(X2|Z). The nonparametric estimator is employed only when the curse of dimensionality in matching variables prevents MSII from attaining parametric convergence. Alternatively, it is possible to use a nonparametric estimate of g2(Z) as a (generated) regressor in place of X2 in regression (1) from the beginning. As illustrated in Section 2.4, this would result in a partially linear semiparametric model with a measurement error problem. There could be several different (nonparametric) estimators available for the model of this class. However, such estimators are not as widely used in practice as the OLS, and hence we leave the development of the estimators for future work.