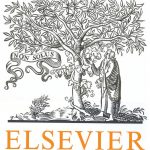
ترجمه مقاله نقش ضروری ارتباطات 6G با چشم انداز صنعت 4.0
- مبلغ: ۸۶,۰۰۰ تومان
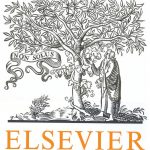
ترجمه مقاله پایداری توسعه شهری، تعدیل ساختار صنعتی و کارایی کاربری زمین
- مبلغ: ۹۱,۰۰۰ تومان
Abstract
Nowadays huges volumes of data are produced in the form of fast streams, which are further affected by non-stationary phenomena. The resulting lack of stationarity in the distribution of the produced data calls for efficient and scalable algorithms for online analysis capable of adapting such changes (concept drift). The online learning field has lately turned its focus on this challenging scenario, by designing incremental learning algorithms that avoid becoming obsolete after a concept drift occurs. Despite the noted activity in the literature, a need for new efficient and scalable algorithms that adapt to the drift still prevails as a research topic deserving further effort. Surprisingly, Spiking Neural Networks, one of the major exponents of the third generation of artificial neural networks, have not been thoroughly studied as an online learning approach, even though they are naturally suited to easily and quickly adapting to changing environments. This work covers this research gap by adapting Spiking Neural Networks to meet the processing requirements that online learning scenarios impose. In particular the work focuses on limiting the size of the neuron repository and making the most of this limited size by resorting to data reduction techniques. Experiments with synthetic and real data sets are discussed, leading to the empirically validated assertion that, by virtue of a tailored exploitation of the neuron repository, Spiking Neural Networks adapt better to drifts, obtaining higher accuracy scores than naive versions of Spiking Neural Networks for online learning environments.
Conclusions and Future Research Lines
This work has presented a portfolio of new adaptations of evolving Spiking Neural Networks (eSNNs) for online learning scenarios under concept drift. Firstly, we have adapted the traditional eSNN technique to be used on online data streams by limiting the size of the neuron repository, yielding the so-called Online eSNN (OeSNN). Secondly, we have embraced the use of selective and generative data reduction techniques (DRTs) to optimize the contents of the neuron repository so as to achieve a better adaptability of the model to changing concepts over the processed data stream. Both passive and active strategies have been defined to incorporate DRTs into the OeSNN learning procedure: the active comprises a drift detector that detects changes along the data stream and triggers the application of the DRT at hand to the neuron repository. Two different families of OeSNN models have been proposed: OeSNN-PS (using prototype-selection DRTs) and OeSNN (corr. using prototype-generation DRTs), both capable of operating in passive and active modes when processing data streams. An extensive set of computer experiments over synthetic and real streaming datasets has been designed and discussed to find performance differences between the above approaches. Part of the OeSNN-PS variants (OeSNN-SMMA and OeSNN-CNN) and all OeSNN-PG approaches (i.e. OeSNN-SGP, OeSNNSGP2, and OeSNN-ASGP) have been proven to attain better predictive scores during plasticity periods than the naive version of the OeSNN (i.e. the proposed OeSNN with no DRT included in its learning algorithm). The application of DRTs to the proposed OeSNN model also allows reducing the required space to store output neurons, thus decreasing the processing time needed to train with newly samples: the less neurons in the repository, the less similarity computations during the learning phase. This alleviation of the computational resources demanded by the model is of utmost importance in online learning, where processing times and storage should be kept as low as possible to process high stream data rates.