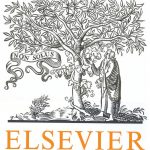
ترجمه مقاله نقش ضروری ارتباطات 6G با چشم انداز صنعت 4.0
- مبلغ: ۸۶,۰۰۰ تومان
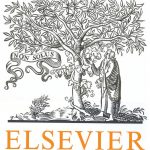
ترجمه مقاله پایداری توسعه شهری، تعدیل ساختار صنعتی و کارایی کاربری زمین
- مبلغ: ۹۱,۰۰۰ تومان
Abstract
Within the large scale classification problem, the stochastic gradient descent method called PEGASOS has been successfully applied to support vector machines (SVMs). In this paper, we propose a stochastic gradient twin support vector machine (SGTSVM) based on the twin support vector machine (TWSVM). Compared to PEGASOS, our method is insensitive to stochastic sampling. Furthermore, we prove the convergence of SGTSVM and the approximation between TWSVM and SGTSVM under uniform sampling, whereas PEGASOS is almost surely convergent and only has an opportunity to obtain an approximation to SVM. In addition, we extend SGTSVM to nonlinear classification problems via a kernel trick. Experiments on artificial and publicly available datasets show that our method has stable performance and can handle large scale problems easily.
Conclusion
An insensitive stochastic gradient twin support vector machine (SGTSVM) has been proposed. This method is less sensitive to sampling than PEGA SOS while having better convergence and approximation. The experimental results have shown that our method has a better performance and a higher training speed than PEGASOS and LIBLINEAR. For practical convenience, the corresponding SGTSVM source code (including programs in Matlab and the C language) have been uploaded to http://www.optimal-group.org/ Resources/Code/SGTSVM.html. The possibilities for future research include designing a special sampling for SGTSVM to obtain a better performance and applying SGTSVM to big data problems.