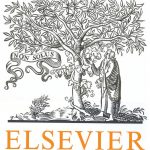
ترجمه مقاله نقش ضروری ارتباطات 6G با چشم انداز صنعت 4.0
- مبلغ: ۸۶,۰۰۰ تومان
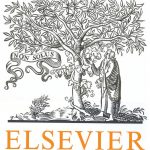
ترجمه مقاله پایداری توسعه شهری، تعدیل ساختار صنعتی و کارایی کاربری زمین
- مبلغ: ۹۱,۰۰۰ تومان
Abstract
With rapid development of financial services and products, credit risk assessment has recently gained considerable attention in the field of financial risk management. In this paper, an improved credit risk assessment approach is presented. Based on the credit data from China Banking Regulatory Commission (CBRC), a multi-dimensional and multi-level credit risk indicator system is constructed. In particular, we present an improved sequential minimal optimization (SMO) learning algorithm, named four-variable SMO (FV-SMO), for credit risk classification model. At each iteration, it jointly selects four variables into the working set and an theorem is proposed to guarantee the analytical solution of sub-problem. The assessment is made on China credit dataset and two benchmark credit datasets from UCI database and CD-ROM database. Experimental results demonstrate FVSMO is competitive in saving the computational cost and outperforms other five state-of-the-art classification methods in credit risk assessment accuracy.
VI. CONCLUSION AND FUTURE WORK
In this paper, we present a novel SMO learning algorithm on a four-variable working set for classification model and applied it to China credit dataset and two benchmark datasets. This method derived by solving a series of the QP sub-problems with four variables and these sub-problems are solved analytically so that the proposed method approaches to the optimal solution more quickly. Numerical results demonstrate that the proposed method has faster speed with statistical significance. Besides, experimental results also illustrate that FV-SMO can get the satisfactory performance in the classification accuracy, which provides compelling evidence of the advantages of FVSMO. Given its encouraging performance, we are aiming to extend the algorithm to solve the problem of multi-class and regression problem instead of the binary classification. Another contribution of this work is the multi-dimensional and multi-level credit risk indicator system. According to our knowledge, it is the first attempt to build the comprehensive indicator system on real credit data of China’s banking. The system can not only help the banking managers and the audience of this paper to understand the overall situation of China’s credit risk, but also scree out the key indicators that should been monitored by the policy makers. For future work, we could explore this system for more credit risk management applications.