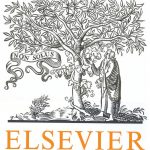
ترجمه مقاله نقش ضروری ارتباطات 6G با چشم انداز صنعت 4.0
- مبلغ: ۸۶,۰۰۰ تومان
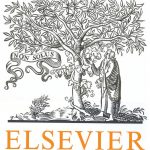
ترجمه مقاله پایداری توسعه شهری، تعدیل ساختار صنعتی و کارایی کاربری زمین
- مبلغ: ۹۱,۰۰۰ تومان
abstract
Speech Emotion Recognition (SER) can be regarded as a static or dynamic classification problem, which makes SER an excellent test bed for investigating and comparing various deep learning architectures. We describe a frame-based formulation to SER that relies on minimal speech processing and end-to-end deep learning to model intra-utterance dynamics. We use the proposed SER system to empirically explore feed-forward and recurrent neural network architectures and their variants. Experiments conducted illuminate the advantages and limitations of these architectures in paralinguistic speech recognition and emotion recognition in particular. As a result of our exploration, we report state-of-the-art results on the IEMOCAP database for speaker-independent SER and present quantitative and qualitative assessments of the models’ performances.
Conclusion
Various deep learning architectures were explored on a Speech Emotion Recognition (SER) task. Experiments conducted illuminate how feed-forward and recurrent neural network architectures and their variants could be employed for paralinguistic speech recognition, particularly emotion recognition. Convolutional Neural Networks (ConvNets) demonstrated better discriminative performance compared to other architectures. As a result of our exploration, the proposed SER system which relies on minimal speech processing and end-to-end deep learning, in a framebased formulation, yields state-of-the-art results on the IEMOCAP database for speaker-independent SER. Future work can be pursued in several directions. The proposed SER system can be integrated with automatic speech recognition, employing joint knowledge of the linguistic and paralinguistic components of speech to achieve a unified model for speech processing. More generally, observations made in this work as a result of exploring various architectures could be beneficial for devising further architectural innovations in deep learning that can exploit advantages of current models and address their limitations.