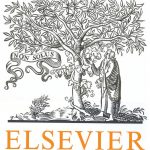
ترجمه مقاله نقش ضروری ارتباطات 6G با چشم انداز صنعت 4.0
- مبلغ: ۸۶,۰۰۰ تومان
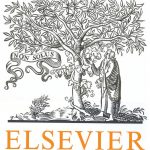
ترجمه مقاله پایداری توسعه شهری، تعدیل ساختار صنعتی و کارایی کاربری زمین
- مبلغ: ۹۱,۰۰۰ تومان
Alert correlation is an important technique for managing large the volume of intrusion alerts that are raised by heterogenous Intrusion Detection Systems (IDSs). The recent trend of research in this area is towards extracting attack strategies from raw intrusion alerts. It is generally believed that pure intrusion detection no longer can satisfy the security needs of organizations. Intrusion response and prevention are now becoming crucially important for protecting the network and minimizing damage. Knowing the real security situation of a network and the strategies used by the attackers enables network administrators to launches appropriate response to stop attacks and prevent them from escalating. This is also the primary goal of using alert correlation technique. However, most of the current alert correlation techniques only focus on clustering inter-connected alerts into different groups without further analyzing the strategies of the attackers. Some techniques for extracting attack strategies have been proposed in recent years, but they normally require defining a larger number of rules. This paper focuses on developing a new alert correlation technique that can help to automatically extract attack strategies from a large volume of intrusion alerts, without specific prior knowledge about these alerts. The proposed approach is based on two different neural network approaches, namely, Multilayer Perceptron (MLP) and Support Vector Machine (SVM). The probabilistic output of these two methods is used to determine with which previous alerts this current alert should be correlated. This suggests the causal relationship of two alerts, which is helpful for constructing attack scenarios. One of the distinguishing feature of the proposed technique is that an Alert Correlation Matrix (ACM) is used to store correlation strengthes of any two types of alerts. ACM is updated in the training process, and the information (correlation strength) is then used for extracting high level attack strategies.
همبستگی هشدار یک روش مهم در مدیریت حجم هشدارهای نفوذ که توسط سیستم های شناسایی نفوذ خارجی تولید می شوند است. روند اخیر تحقیقات در این زمینه به سمت استخراج استراتژی های حمله از هشدارهای نفوذ اولیه است. به طور کلی باور شده است که شناسایی نفوذ خالص بیشتر ازین نمی تواند نیازهای امنیتی سازمان ها را براورده کند. اکنون واکنش نفوذ و پیشگیری همیت قاطع برای حفاظت شبکه و کهش خطرات دارند. شناختن موقیت امنیتی واقعی یک شبکه و استراتژی استفاده شده توسط مهاجمان، مدیر شبکه را قادر می سازد که واکنش مناسب برای توقف حملات انجام دهد و از افزایش آنها جلوگیری کند. این هدف اولیه روش های همبستگی هشدار فعلی است. گرچه بیشترین روش های همبستگی هشدار فعلی فقط روی دسته بندی هشدارهای اتصال داخلی به گروه های متفاوت بدون تحلیل بیشتر استراتژی های حملات، تمرکز می کند. تعدادی از روش ها برای استخراج استراتژی های حمله در سال های اخیر پیشنهاد شده اند اما به طور نرمال نیازمند تعریف یک تعداد بزرگتر قوانین هستند. این مقاله بر توسعه یک روش همبستگی هشدار جدید تمرکز دارد که می تواند به استخراج خودکار استراتژی های حمله در یک حجم بزرگ هشدارهای نفوذ، بدون دانش قبلی ویژه درمورد این هشدارها، کمک کند. روش پیشنهادی بر اساس دو روش شبکه عصبی متفاوت است به نام (Multilayer Perceptron (MLP و (Support Vector Machine (SVM . خروجی احتمالی این دو روش برای تعیین اینکه کدام هشدار باید واسته باشد به کار می رود. این رابطه علی دو هشدار را پیشنهاد می دهد که برای ساخت سناریو های حمله مفید است. یکی از مشخصه های اختصاصی روش پیشنهادی این است که یک ماتریس همبستگی هشدار (ACM) برای ذخیره نقاط قوت همبستگی هر دو نوع از هشدارها استفاده می شود. ACM در پروسه آموزش به روز رسانی می شود و اطلاعات (قدرت همبستگی) برای استخراج استراتژی های حمله سطح بالا استفاده می شود.