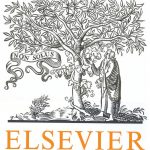
ترجمه مقاله نقش ضروری ارتباطات 6G با چشم انداز صنعت 4.0
- مبلغ: ۸۶,۰۰۰ تومان
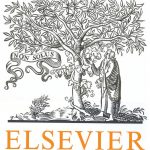
ترجمه مقاله پایداری توسعه شهری، تعدیل ساختار صنعتی و کارایی کاربری زمین
- مبلغ: ۹۱,۰۰۰ تومان
This paper investigates reactive elasticity in stream processing environments where the performance goal is to analyze large amounts of data with low latency and minimum resources. Working in the context of Apache Storm, we propose an elastic management strategy which modulates the parallelism degree of applications' components while explicitly addressing the hierarchy of execution containers (virtual machines, processes and threads). We show that provisioning the wrong kind of container may lead to performance degradation and propose a solution that provisions the least expensive container (with minimum resources) to increase performance. We describe our monitoring metrics and show how we take into account the specifics of an execution environment. We provide an experimental evaluation with real-world applications which validates the applicability of our approach.
1 INTRODUCTION
BIg data is a challenge in various computing system domains. It is present in IoT with the proliferation of connected devices, grows with the increasing scale of high performance computing systems and is coupled with the expanding Internet and social network activities. It is a major topic in the data intelligence business.
There are two major techniques to process big data: batch processing and stream processing. In batch processing, data is first stored in huge databases and is processed later, usually with scalable programming models such as Google’s MapReduce [1]. However, with the ever growing size of data, the cost of data transfer and storage becomes prohibitive [2], [3]. Moreover, in multiple domains, what is important is not to keep the initial data but to analyze it as fast as possible to produce valuable intelligence [4], [5]. To tackle these issues, stream processing systems put the emphasis on reactivity and analyze data as it is produced. Recent years have seen the emergence of multiple stream processing solutions [6], [7], [8], [9].
7 CONCLUSION
The focus of our paper is on the impact of different execution containers on the performance of an elastic stream processing system. We have explicitly considered the hierarchy of execution containers (machines, processes and threads) and have shown that their provisioning comes at a different cost. More importantly, we have shown that provisioning the wrong type of containers may decrease performance.
1. مقدمه
داده های بزرگ یک چالش در حوزه های مختلف سیستم محاسباتی است. داده های بزرگ در اینترنت اشیاء نیز وجود دارد و با تکثیر دستگاه های متصل، همراه با مقیاس فزاینده سیستم های کامپیوتری با عملکرد بالا رشد میکند و با فعالیت های اینترنتی و شبکه های اجتماعی رو به رشد، همراه است. این یک موضوع اصلی در کسب و کار هوش داده ای است.
دو تکنیک اصلی برای پردازش داده های بزرگ وجود دارد: پردازش دسته ای و پردازش جریانی. در پردازش دسته ای، داده ها ابتدا در پایگاه داده های بزرگی ذخیره میشوند و بعداً پردازش می گردند؛ اینکار معمولاً با مدلهای برنامه نویسی مقیاس پذیری مانند Google’s MapReduce انجام میشود. با اینحال با اندازه رو به رشد داده ها، هزینه انتقال و ذخیره سازی آنها قابل جلوگیری نیست. بعلاوه در بسیاری از دامنه ها، چیزی که مهم است نگه داشتن داده های اولیه نیست بلکه تحلیل آنها در سریعترین زمان ممکن است تا اطلاعات ارزشمندی را ایجاد کنند. سیستم های پردازش جریانی برای حل این مسائل، بر واکنش پذیری و تحلیل داده ها به محض تولید شدن آنها، تأکید دارند. سالهای اخیر شاهد پیدایش راه حل های مختلفی از پردازش جریانی بوده است.
7. نتیجه گیری
تمرکز مطالعه ما بر روی تأثیر محفظه های اجرایی مختلف بر عملکرد یک سیستم پردازش جریانی ارتجاعی است. ما سلسله مراتب محفظه های اجرایی (ماشین ها، فرآیندها و نخ ها) را بصورت واضح بررسی کرده ایم و نشان داده ایم که فراهم سازی آنها با هزینه های مختلفی انجام می شود. مهمتر اینکه ما نشان داده ایم که فراهم سازی نوع اشتباهی از محفظه ها میتواند سبب افت عملکرد گردد.