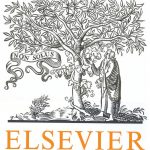
ترجمه مقاله نقش ضروری ارتباطات 6G با چشم انداز صنعت 4.0
- مبلغ: ۸۶,۰۰۰ تومان
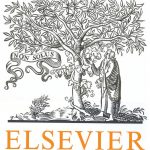
ترجمه مقاله پایداری توسعه شهری، تعدیل ساختار صنعتی و کارایی کاربری زمین
- مبلغ: ۹۱,۰۰۰ تومان
In this work we propose the GLRT-MP algorithm which combines compressed sensing techniques and classical detection theory and explores its application to sparse arrays. Sparse arrays are large undersample arrays with nonuniform spacing that provides high resolution at the cost of high sidelobes. Compressed sensing techniques are able to minimize the undesired effects of the large array, while classical detection theory provides a way to perform detection while maintaining a desired false alarm probability. We provide analysis of the GLRT when the noise power is known and unknown, the latter which will allow one to design a CFAR radar. We provide numerical results to verify our results.
I. INTRODUCTION
It is well known that a uniform linear array (ULA) with half wavelength spacing ensures the absence of grating lobes in the visible region of the array pattern. ULAs also produce low sidelobes, a desirable trait during the beamforming stages of target detection. In contrast, sparse arrays with large, nonuniform inter-element spacing produces large sidelobes but achieve higher resolution due to the large aperture [16]. However, due to the large sidelobes, the beamformer often experience false peaks, which increases the probability of false alarm.
In recent years, compressive sensing (CS) techniques tailored for sparse localization frameworks [4], [8] were shown to be able to cope with the spatial undersampling of sparse arrays [5]. This exciting result enables the radar designer to implement large undersampled arrays without needlessly increasing the false alarm probability. However, much of the literature in CS techniques addressing localization of sparse targets (e.g. [9], [10], [11]) fails to explain how CS techniques affect parameters of interest to radar, specifically the probabilities of false alarm and detection.
V. CONCLUSIONS
In this paper, we developed a GLRT for multiple targets when the noise power is known and provided the false alarm and detection probabilities. We then build upon the known noise case and develop a CFAR detector for multiple targets and provide the false alarm and detection probabilities. We then propose the GLRT-MP algorithm, a detector that combines matching pursuit concepts and the GLRT derived in this paper. Numerical results show that the analytical expressions derived in this paper closely match the simulation results and outperforms the conventional beamformer.
1- مقدمه
به خوبی مشخص است که یک آرایه خطی همگن (ULA) با فاصله بندی نصف طول موج، عدم وجود لوب های گریتینگ در نواحی قابل مشاهده الگوی آنتن را تضمین می کند. ULA ها همچنین لوب های جانبی پایین که ویژگی مطلوبی در حین مراحل بیم فورمینگ (شکل دهی گلبرگ آنتن) در تشخیص هدف می باشد، فراهم می کنند. آرایه های اسپارس با فاصله بندی اجزای داخلی غیر همگن، لوب های جانبی بزرگ ایجاد کرده اما به علت داشتن دهانه بزرگ دست یابی به رزولوشن بیشتر را ممکن می سازند. اما به علت وجود لوب های جانبی بزرگ، بیم فورمر (شکل دهنده ی بیم) اغلب دچار پیک های اشتباه می شود که احتمال هشدار اشتباه را افزایش می دهد.
در سال های اخیر تکنیک های سنجش فشرده (CS) که برای فریم ورک های های تعیین موقعیت اسپارس ساخته شده اند، نشان داده اند که قادر به مقابله با آندر سمپلینگ آرایه های اسپارس هستند. این نتایج جالب، طراحان آنتن را قادر به پیاده سازی آرایه های آندر سمپل بزرگ می سازد بدون اینکه احتمال هشدار اشتباه افزایش یابد. اما بیشتر مقالات در مورد تکنیک های CS که به بررسی تعیین محل اهداف اسپارس می پردازند، در توضیح این که چگونه تکنیک های CS روی پارامتر های مورد نظر آنتن تاثیر گذاشته خصوصا احتمال هشدار و تشخیص اشتباه، دچار مشکل هستند.
5- نتیجه گیری
ما در این مقاله یک GLRT برای اهداف چندگانه زمانی که قدرت نویز معلوم است توسعه داده و احتمال تشخیص و هشدار اشتباه را برای آن ارائه دادیم. سپس همین موارد را برای حالت نویز نامعلوم انجام داده و یک حسگر CFASR برای اهداف چندگانه توسعه داده و احتمال تشخیص و هشدار اشتباه را برای آن ارائه دادیم . سپس الگوریتم GLRT-MP را پیشنهاد کرده که حسگری است که مفاهیم دنبال کردن تطابق و GLRT موجود در این مقاله را با هم ترکیب می کند. نتایج عددی نشان می دهد که عبارات اماری موجود دراین مقاله به نتایج شبیه سازی بسیار نزدیک بوده و عملکرد بهتری نسبت به بیم فورمر سنتی دارد.