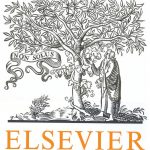
ترجمه مقاله نقش ضروری ارتباطات 6G با چشم انداز صنعت 4.0
- مبلغ: ۸۶,۰۰۰ تومان
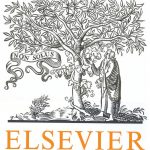
ترجمه مقاله پایداری توسعه شهری، تعدیل ساختار صنعتی و کارایی کاربری زمین
- مبلغ: ۹۱,۰۰۰ تومان
و برای خرید ترجمه کامل مقاله با فرمت ورد از دکمه های زیر استفاده نمایید.
In this paper, we use artificial intelligence image recognition to obtain Digestive endoscopy image, and process the image based on 5G Deep learning edge algorithm to judge the disease type of the patient, and then consider the treatment plan. The combination of body area network and edge computing technology can meet the demand of low delay in body area network. In this case, the resource constrained body area network gateway node can process the physiological data collected by it into an offloadable task, and then unload the task and data to the edge computing node according to a certain strategy. The edge computing node completes the corresponding task processing and data storage, and finally provides the results to the relevant medical institutions and body area network users for reading auxiliary diagnosis and treatment of diseases. Studies have shown that 25% of patients with colon polyps have CD4 cells in peripheral blood based on 5G deep learning edge algorithm under artificial intelligence image recognition of Digestive endoscopy. The number of lymphocyte in group of differentiation was less than 200/μL, and the blood RNA in 92.3% patients was lower than 100 IU/ml, while fam CTP (A-cyclic peptide) was lower than 100 IU/ml. Opportunistic infections of the intestine and viruses can directly cause enteropathy because the fluorescence intensity of the probe is essentially unchanged and cannot form a triple helix structure. In terms of feature recognition accuracy, the 5G deep learning edge algorithm in this paper improves accuracy by 68% compared to the simple Yolo algorithm, and is similar in speed. Compared with RCNN algorithm, the accuracy and speed are improved by 21% and 85% respectively. Therefore, the 5G deep learning edge algorithm based on artificial intelligence image recognition has the advantages of accuracy and speed in digestive endoscopy of intelligent medical.
1. Introduction
Smart medicine is a hot topic in recent years, which is a new interdisciplinary subject integrating life science and information science. There is no very clear definition of smart medicine. The mainstream view is that it is based on medical informatization. The core is to manage patient identities through the Internet of Things and sensor technology and form patient indexes in hospital information systems. Based on this, information exchange and communication are performed according to business logic and network protocols to enable intelligent identification and identification location, tracking, monitoring and management.
5. Conclusions
According to the medical characteristics of intelligent medical human body tomography, the original OCR image is divided into four blocks, and each sub block reflects the local characteristics of different directions. A single signal is used to describe the spectral and local features of each subblock and build a feature vector. A single feature vector of the four subblocks is categorized by the SRC to get the reconstruction error vector. The four subblock reconstruction error vectors are weighted and fused based on a random weighting matrix. Through statistical analysis of the fusion results of multiple groups of weight vectors, the decision variables are designed and sample categories are obtained. Four test conditions were set in MSTAR dataset, including standard operating conditions and extended operating conditions. Strengthening the construction of medical humanities may help us to arouse our self-contained medical emotions and wisdom, guide us to understand the truth behind medicine, get rid of blind adherence and superstition to medical science and technology, and return to the original nature of medicine. The main body is not only the medical staff, but also the patients and their families. This is very suitable to start with the intelligent medical treatment of hypertension, which has become a public problem. With the help of the intelligent medical treatment of digestive endoscopy, it is the main topic of general practice in the future to realize the intelligent medical treatment of more diseases.
در این مقاله از تشخیص تصویر هوش مصنوعی برای بدست آوردن تصویر آندوسکوپی گوارشی استفاده می کنیم، و تصویر را براساس الگوریتم لبه یادگیری عمیق 5G پردازش می کنیم تا درباره نوع بیماری قضاوت کنیم و سپس برنامه درمانی را در نظر بگیریم. ترکیب شبکه ناحیه بدنی و فناوری رایانش لبه می تواند تقاضایی با تاخیر کم را در شبکه ناحیه بدن برآورده کند. در این مورد، گره گیت وی شبکه ناحیه بدنی محدود شده با منابع می تواند داده های فیزیولوژیکی جمع آوری شده را با یک وظیفه قابل بارگیری پردازش کند، و سپس با توجه به یک استراتژی خاص، وظایف و داده ها را در گره محاسباتی لبه تخلیه کند. گره رایانش لبه، پردازش مرتبط با وظیفه و ذخیره سازی داده را تکمیل می کند، و در نهایت نتایج را به موسسات پزشکی مربوطه و کاربران شبکه ناحیه بدنی برای خواندن تشخیص کمکی و درمان بیماری ها ارائه می دهد. تحقیقات نشان داده اند که براساس الگوریتم لبه یادگیری عمیق 5G تحت تشخیص تصویر هوش مصنوعی آندوسکوپی گوارشی، 25% بیماران مبتلا به پولیپ روده دارای سلول های CD4 در خون محیطی هستند. تعداد لنفوسیت ها در گروه تمایز کمتر از 200 μL بود، و RNA خونی در 92.3% بیماران کمتر از 100IU/ml بود، در حالی که CTP فام (پپتید A-حلقه ای) کمتر از 100 IU/ml بود. عفونت های فرصت طلب روده و ویروس ها می توانند به صورت مستقیم باعث آنتروپاتی شوند زیرا شدت فلورسنس پروب در اصل بدون تغییر باقی می ماند و نمی تواند یک ساختار مارپیچ سه گانه را شکل دهد. الگوریتم لبه یادگیری عمیق 5G در مقایسه با الگوریتم ساده یولو از نظر دقت تشخیص ویژگی در این مقاله، دقت را تا 68% بهبود می دهد و از نظر سرعت مشابه است. دقت و سرعت به ترتیب در مقایسه با الگوریتم RCNN تا 21% و 85% بهبود می یابند. بنابراین، الگوریتم لبه یادگیری عمیق 5G مبتنی بر تشخیص تصویر هوش مصنوعی دارای مزایای دقت و سرعت در آندوسکوپی گوارشی پزشکی هوشمند است.
1. مقدمه
پزشکی هوشمند یکی از موضوعات داغ در سالهای اخیر است که یک موضوع جدید بین رشته ای است که علم زندگی و علم اطلاعات را ادغام می کند. هیچ تعریف واضحی از پزشکی هوشمند وجود ندارد. دیدگاه اصلی این است که مبتنی بر اطلاع رسانی پزشکی است. هسته اصلی، مدیریت هویت بیماران از طریق اینترنت اشیا و فناوری حسگر و تشکیل نمایه های بیمار در سیستم های اطلاعات بیمارستان است. بر این اساس، تبادل اطلاعات و ارتباطات با توجه به منطق کسب و کار و پروتکل های شبکه انجام می شود تا شناسایی هوشمند و مکان شناسایی، ردیابی، پایش و مدیریت را ممکن سازد.
5. نتیجه گیری ها
با توجه به ویژگی های پزشکی توموگرافی هوشمند پزشکی بدن انسان، تصویر اصلی OCR به چهار بلوک تقسیم میشود، و هر بلوک فرعی نشان دهنده ویژگی های محلی جهت های مختلف است. یک سیگنال واحد برای توصیف ویژگی های محلی و طیفی هر بلوک فرعی استفاده می شود و یک بردار ویژه ساخته می شود. یک بردار ویژه منحصر به فرد از چهار بلوک فرعی توسط SRC دسته بندی می شود تا بردار خطای بازسازی به دست آید. چهار بردار خطای بازسازی بلوک فرعی وزن دهی میشوند و بر اساس یک ماتریس تصادفی وزن دهی ادغام می شوند. از طریق تحلیل آماری نتایج ادغام چندین گروه از بردارهای وزنی متغیرهای تصمیم طراحی میشوند و دستههای نمونه به دست می آیند. چهار شرط آزمون در مجموعه داده های MSTAR از جمله شرایط عملیاتی استاندارد و شرایط عملیاتی قابل تعمیم تعیین میشوند. تقویت ساختار علوم انسانی پزشکی به ما کمک می کند تا احساسات و خرد پزشکی خود را بیدار کنیم و ما را به درک حقیقت پشت پزشکی، رهایی از پایبندی کورکورانه و خرافات به علم و فناوری پزشکی، و بازگشت به ماهیت اصلی پزشکی هدایت می کند. مجموعه اصلی تنها کارکنان پزشکی نیستند، بلکه بیماران و خانوادههای آنها هستند. درمان پزشکی هوشمند فشار خون که به یک مشکل عمومی تبدیل شده است، برای شروع بسیار مناسب است. با کمک درمان هوشمند پزشکی آندوسکوپی گوارشی، تحقیق درمان هوشمند پزشکی بیشتر بیماریها موضوع اصلی روش عمومی در آینده است.